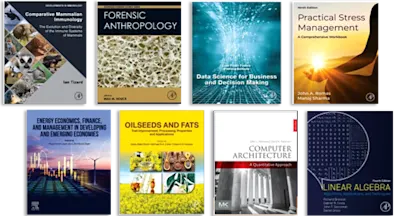
Computational Intelligence and Modelling Techniques for Disease Detection in Mammogram Images
- 1st Edition - November 16, 2023
- Imprint: Academic Press
- Editor: D. Jude Hemanth
- Language: English
- Paperback ISBN:9 7 8 - 0 - 4 4 3 - 1 3 9 9 9 - 4
- eBook ISBN:9 7 8 - 0 - 4 4 3 - 1 4 0 0 0 - 6
Computational Intelligence and Modelling Techniques for Disease Detection in Mammogram Images comprehensively examines the wide range of AI-based mammogram analysis methods f… Read more
Purchase options
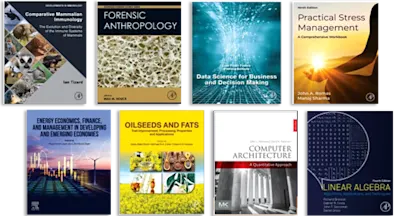
Computational Intelligence and Modelling Techniques for Disease Detection in Mammogram Images comprehensively examines the wide range of AI-based mammogram analysis methods for medical applications. Beginning with an introductory overview of mammogram data analysis, the book covers the current technologies such as ultrasound, molecular breast imaging (MBI), magnetic resonance (MR), and Positron Emission mammography (PEM), as well as the recent advancements in 3D breast tomosynthesis and 4D mammogram. Deep learning models are presented in each chapter to show how they can assist in the efficient processing of breast images.
The book also discusses hybrid intelligence approaches for early-stage detection and the use of machine learning classifiers for cancer detection, staging and density assessment in order to develop a proper treatment plan. This book will not only aid computer scientists and medical practitioners in developing a real-time AI based mammogram analysis system, but also addresses the issues and challenges with the current processing methods which are not conducive for real-time applications.
- Presents novel ideas for AI based mammogram data analysis
- Discusses the roles deep learning and machine learning techniques play in efficient processing of mammogram images and in the accurate defining of different types of breast cancer
- Features dozens of real-world case studies from contributors across the globe
Graduate students, researchers and professionals in the fields of computational intelligence, bioinformatics, and biomedical engineering, Medical Researchers, Medical Libraries, Medical Practitioners, Government and Non-government organizations
2. AI in Breast Imaging: Applications, Challenges and Future Research
3. Prediction of Breast Cancer Diagnosis Using a Random Forest Classifier
4. Medical Image Analysis of masses in Mammography using Deep Learning model for Earlier Diagnosis of Cancer Tissues
5. A framwork for breast cancer diagnostics based on MobileNetV2 and LSTM-based deep learning
6. Autoencoder based dimensionality reduction in 3D breast images for efficient classification with processing by deep learning architectures
7. Prognosis of breast cancer using machine learning classifiers
8. Breast cancer diagnosis through microcalcification
9. Scutinization of Mammogram Images using deep learning
10. Computational Techniques for Analysis of Breast Cancer Using Molecular Breast Imaging
11. Machine learning and deep learning techniques for breast cancer detection using ultrasound imaging
12. Efficient Transfer Learning Techniques for Breast Cancer Histopathological Image Classification
13. Classification of breast cancer histopathological images based on shape and texture attributes with ensemble machine learning methods
14. An automatic level set segmentation of breast Tumor from mammogram images using optimized Fuzzy c-means clustering
- Edition: 1
- Published: November 16, 2023
- Imprint: Academic Press
- Language: English
DH