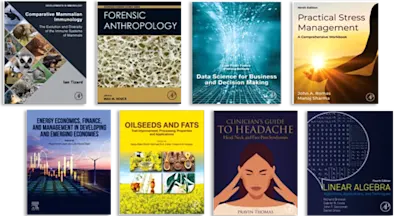
Computational Biology for Stem Cell Research
- 1st Edition - January 12, 2024
- Imprint: Academic Press
- Editors: Pawan Raghav, Rajesh Kumar, Anjali Lathwal, Navneet Sharma
- Language: English
- Paperback ISBN:9 7 8 - 0 - 4 4 3 - 1 3 2 2 2 - 3
- eBook ISBN:9 7 8 - 0 - 4 4 3 - 1 3 2 2 1 - 6
Computational Biology for Stem Cell Research seamlessly bridges the gap between the worlds of biomedical sciences and in silico computational methods. This book serves as a… Read more
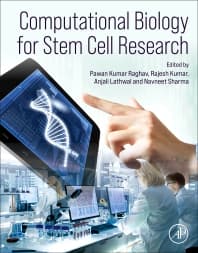
Purchase options
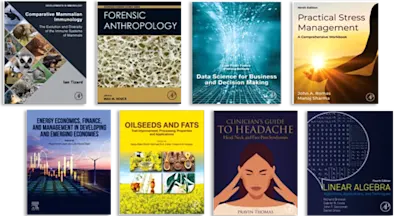
Institutional subscription on ScienceDirect
Request a sales quoteComputational Biology for Stem Cell Research seamlessly bridges the gap between the worlds of biomedical sciences and in silico computational methods. This book serves as a valuable resource for researchers and students, enabling them to grasp and delve into the intricacies of hematopoietic Stem Cells (HSCs) and mesenchymal Stem Cells (MSCs) through the lens of computational biology. This perspective sheds light on stem cell transplantation, translational research, and unique properties of stem cells like self-renewal and differentiation. In addition to introducing readers to stem cell-focused bioinformatics tools, this resource offers a clear pathway for effortlessly merging in silico methods with traditional in vitro and in vivo approaches.
Computational Biology for Stem Cell Research combines science and technology to showcase how computational methods transform stem cell research by reducing costs and enhancing investigations. The chapters uncover various approaches, from machine learning to genome analysis, for studying networks, protein interactions, dynamics, and the preprocessing of large datasets. The book aims to give readers a broad view of the advanced computational tools and methods extensively employed in stem cell research. Additionally, the book emphasizes the ongoing studies and tools yet to be developed for furthering stem cell research.
- Modeling Stem Cell Behavior: Explore stem cell behavior through animal models, bridging laboratory studies to real-world clinical allogeneic HSC transplantation (HSCT) scenarios.
- Bioinformatics-Driven Translational Research: Navigate a path from bench to bedside with cutting-edge bioinformatics approaches, translating computational insights into tangible advancements in stem cell research and medical applications.
- Interdisciplinary Resource: Discover a single comprehensive resource catering to biomedical sciences, life sciences, and chemistry fields, offering essential insights into computational tools vital for modern research.
- Cover image
- Title page
- Table of Contents
- Copyright
- Dedication
- Abbreviations
- List of figures
- List of tables
- Contributors
- Biographies
- Preface
- Acknowledgments
- Introduction
- Section I. In silico tools and approaches in stem cell biology
- Chapter 1. Advancement of in silico tools for stem cell research
- 1. Introduction
- 2. In silico tools and approaches
- 3. Conclusion and future prospects
- Chapter 2. Paradigm shift in stem cell research with computational tools, techniques, and databases
- 1. Introduction
- 2. Big data in stem cell research
- 3. Computational methods for effective analyses of high-throughput stem cells data
- 4. Computational resources for stem cell data analysis
- 5. Cancer stem cells
- 6. Conclusion
- Chapter 3. Stem cell informatics: Web resources aiding in stem cell research
- 1. Introduction
- 2. Discussion
- 3. Conclusion
- Chapter 4. Stem cell based informatics development and approaches
- 1. Introduction
- 2. Applications of stem cells: a promise to behold
- 3. Expanding horizons of omics and advent of informatics
- 4. Network biology and stem cell informatics
- 5. Recent developments in stem cell informatics
- 6. Future of the stem cell informatics
- Chapter 5. Exploring imaging technologies and computational resources in stem cell research for regenerative medicine: A comprehensive review
- 1. Introduction
- 2. Ideal requisite for molecular imaging
- 3. Imaging strategies employed in regenerative medicine
- 4. Imaging methods using nuclear medicine
- 5. Software programs used in stem cell research
- 6. Current challenges in regenerative medicine imaging
- 7. Artificial intelligence in stem cell imaging
- 8. Future of stem cell imaging with artificial intelligence
- 9. Conclusion
- Chapter 6. Application of machine learning–based approaches in stem cell research
- 1. Introduction
- 2. Types of stem cells and their therapeutic applications
- 3. Overview of machine learning–based model building
- 4. Applications of machine learning in stem cell–based therapies
- 5. Applications of deep learning in stem cell–based therapies
- 6. Evolution of nature-inspired computing and its applications in stem cell–based therapies
- 7. Conclusion and future aspects
- Chapter 7. Stem cell therapy in the era of machine learning
- 1. Introduction
- 2. Current state of stem cell therapies
- 3. Machine learning applications in different biological and medicine fields
- 4. Current trends in machine learning–based stem cell therapy
- 5. Conclusion and future prospects
- Chapter 8. Computational and stem cell biology: Challenges and future perspectives
- 1. Introduction
- 2. Current challenges and contests: Crossing hurdles in stem cell and computational biology
- 3. Disease modeling and computational biology: Current challenges
- 4. Differentiating stem cells, desired cell types, and organoids: Challenges and future aspects
- 5. Future prospective and outlook
- 6. Conclusion
- Section II. Application of genomic and proteomic approaches in stem cell research
- Chapter 9. Single-cell transcriptome profiling in unraveling distinct molecular signatures from cancer stem cells
- 1. Introduction
- 2. Cancer stem cells
- 3. Single-cell transcriptomics
- 4. Single-cell data analysis workflow
- 5. Single-cell studies on detecting distinct molecular signature in cancer
- 6. Future perspectives
- Chapter 10. The single-cell big data analytics: A game changer in bioscience
- 1. Introduction
- 2. Techniques for producing single-cell big data in revolutionizing regenerative medicine healthcare
- 3. Unlocking the potential of stem cells through single-cell data analysis
- 4. The promising future of single-cell data in advancing stem cell biology research
- 5. Conclusion
- Chapter 11. Unravelling the genomics and proteomics aspects of the stemness phenotype in stem cells
- 1. Introduction
- 2. Genomic and proteomic aspects to understand stem cell phenotype
- 3. Mechanistic insights into the stemness phenotype of stem cells through genomic and proteomic platform
- 4. Conclusion
- Chapter 12. Cutting-edge proteogenomics approaches to analyze stem cells at the therapeutic level
- 1. Stem cell characterization
- 2. Conclusion
- Chapter 13. Cheminformatics, metabolomics, and stem cell tissue engineering: A transformative insight
- 1. Introduction
- 2. Computational tools and stem cell biology
- 3. Computational approaches, genomics and proteomics
- 4. Cheminformatics, tissue engineering, and stem cell research
- 5. Metabolomics data acquisition and preprocessing
- 6. Manipulation of stem cells and tissue regeneration
- 7. Stem cell microenvironment and cardiac tissue regeneration: engineered approaches
- 8. Future prospective and present outlook
- 9. Conclusion
- Chapter 14. Advances in regenerative medicines based on mesenchymal stem cell secretome
- 1. Introduction
- 2. MSC-Secretome: conditioned media from mesenchymal stem cells and exosomes
- 3. Effector biological functions of MSC-Secretome and their applications
- 4. Clinical studies with secretome from mesenchymal stem cells
- 5. Applications of computational tools in stem cell–based therapies
- 6. Conclusion
- Chapter 15. Paradigms of omics in bioinformatics for accelerating current trends and prospects of stem cell research
- 1. Introduction
- 2. A brief overview of current therapeutic uses of stem cell treatment
- 3. Characterization of stem cells using OMICS
- 4. Transcriptomic and proteomic methods in stem cell characterization
- 5. Multiomics collaboration
- 6. Potency, omics approaches, and knowledge of the mechanism of action
- 7. Importance of computational modeling in stem cell research
- 8. Research on stem cells: applications of several modeling types
- 9. Bioinformatics in stem cell research
- 10. Stem cell research mechanistic modeling frameworks
- 11. Types of modeling in stem cell research
- 12. Computational modeling applications in regenerative medicine
- 13. Omics-based methods for managing the manufacturing of biomaterials
- 14. Challenges in computational analysis in stem cell research
- 15. Conclusion
- Chapter 16. Transcriptomic profiling–based identification of biomarkers of stem cells
- 1. Introduction
- 2. Timeline of main milestones in technologies for gene expression
- 3. Working methodology
- 4. Various platforms and strategies in RNA-Seq
- 5. Conclusion and future perspectives
- Chapter 17. Genomic and transcriptomic applications in neural stem cell therapeutics
- 1. Introduction
- 2. Genomic approaches to study stem cells
- 3. Genomic methods in therapeutic application of neural stem cells
- 4. Transcriptomics
- 5. Bottlenecks in -omics application in stem cell model for neurological disorders
- Section III. Stem cell network modeling and systems biology
- Chapter 18. Integration of multi-omic data to identify transcriptional targets during human hematopoietic stem cell erythroid differentiation
- 1. Introduction
- 2. Rationale
- 3. Experimental approach
- 4. Bioinformatic pipelines for analyzing transcriptional regulation
- 5. Uncovering GATA-bound regulatory regions: GATA1 and GATA2 ChIP-seq data integration reveals stage-specific TF switching during erythroid differentiation
- 6. Conclusion and future directions—a therapeutic approach
- Chapter 19. Computational approaches to determine stem cell fate
- 1. Introduction
- 2. Understanding stem cell fates during differentiation: new technologies—software and models
- 3. Rise of gene regulatory networks: nodes, motifs, gene batteries, and mathematical models
- 4. Conclusion: future of stem cell engineering applications
- Chapter 20. Stem cell databases and tools: challenges and opportunities of computational biology
- 1. Introduction
- 2. Applications of computational biology for stem cells research
- 3. Stem cell databases
- 4. Cancer stem cell databases
- 5. Resources
- 6. Stem cell tools
- 7. Conclusion and future prospects
- Chapter 21. Deciphering the complexities of stem cells through network biology approaches for their application in regenerative medicine
- 1. Introduction
- 2. Key genes and pathways regulating pluripotent stem cell fate
- 3. Bioinformatics in stem cell research
- Case study: Network analysis using Cytoscape for human pluripotent stem cells
- Chapter 22. Bioinformatics approaches to the understanding of Notch signaling in the biology of stem cells
- 1. Introduction
- 2. Notch signaling
- 3. Applications of bioinformatics in signaling pathways
- 4. Bioinformatics approach in the understanding of Notch signaling in the biology of stem cells
- 5. Clinical studies
- 6. Conclusion
- Chapter 23. In silico approaches for the analysis of developmental fate of stem cells
- 1. Introduction
- 2. Computational techniques in stem and development biology
- 3. Stem cell biology with OMICs
- 4. Epigenetic memory and induced pluripotent stem cells
- 5. Stem cell biology through computation
- 6. Single cell genomics with spatial resolution
- 7. Mathematical modeling
- 8. Weighted correlation network analysis
- 9. Gene set enrichment analysis
- 10. Machine learning classifiers
- 11. Conclusion
- Chapter 24. Computational approaches for hematopoietic stem cells: Advancing regenerative therapeutics
- 1. Introduction
- 2. Big data analysis of HSCs
- 3. Metabolic cross-talk
- 4. Computational approaches for stem cell research
- 5. Computational biology in HSCT
- 6. Major concerns and drawbacks
- 7. Conclusion and future perspectives
- Chapter 25. Approaches to construct and analyze stem cells regulatory networks
- 1. Introduction
- 2. Machine learning methods for stem cell regulatory network prediction
- 3. Toward multiomics data integration for stem cell networks
- 4. Constructing the stem cell gene coexpression networks
- 5. Constructing the stem cell regulatory networks
- 6. Studies using integrated bioinformatics and network-based approaches in stem cells research
- 7. Conclusion and future perspectives
- Section IV. Computational approaches for stem cell tissue engineering
- Chapter 26. Tissue engineering in chondral defect
- 1. Introduction
- 2. Cartilage structure and signaling
- 3. Signaling in tissue engineering
- 4. Pathophysiology of chondral defects and degeneration
- 5. Management of chondral injuries
- 6. Diagnosis of chondral defects
- 7. Management of chondral defects
- 8. Molecular methods of chondral defects management
- 9. Computational tissue engineering
- 10. Limitations
- 11. Conclusions
- Chapter 27. Recent advances in computational modeling: An appraisal of stem cell and tissue engineering research
- 1. Introduction
- 2. The node of computational stem cell biology
- 3. A brief introduction to common modeling approaches
- 4. Types of stem cell-based modeling
- 5. Single-cell isolation-related tools and software
- 6. Multiscale computational modeling: from intracellular networks to cell-cell interactions in the tissue
- 7. Frameworks for mechanistic modeling in stem cell research: an overview
- 8. Computational modeling associated with stem cells in the era of single-cell big data production
- 9. Current obstacles and future possibilities
- 10. SWOT analysis of computational approaches
- 11. Overview of cell modeling: from basic 2D to 3D innovative system
- 12. Computer-aided tissue engineering and regenerative medicines
- 13. Bio-CAD
- 14. Biomimetic designing
- 15. Constriction of stem cell-based tissue-engineered patient-specific implants
- 16. Application of computational modeling to regenerative medicine
- 17. Concluding remarks
- 18. Future prospects
- Chapter 28. Computational approaches for bioengineering of cornea
- 1. Introduction
- 2. Ultrastructure of the cornea
- 3. Corneal diseases
- 4. Corneal transplantation
- 5. Biomaterials for corneal bioengineering
- 6. Computer-aided tissue engineering
- 7. Conclusion
- 8. Future prospective
- Chapter 29. Targeting cancer stem cells and harnessing of computational tools offer new strategies for cancer therapy
- 1. Introduction
- 2. Targeting cancer stem cells for therapy
- 3. Reactive oxygen species and sources
- 4. Reactive oxygen species level in cancer stem cells and drug resistance
- 5. Cancer stem cell for secretion and delivery of anticancer drugs
- 6. Issues of secondary cancer
- 7. In silico modeling of drug development for cancer treatment
- 8. In silico trial methods for anticancer drugs
- 9. Cancer driver genes and computational methods
- 10. Developing new cancer therapies
- 11. Prospects for anticancer drug screening
- 12. Conclusion
- Chapter 30. Introduction to machine learning and its applications in stem cell research
- 1. Introduction
- 2. Introduction to machine learning
- 3. Applications of deep learning in stem cell research
- 4. Discussion
- 5. Challenges and future directions
- Chapter 31. Multiscale computational and machine learning models for designing stem cell-based regenerative medicine therapies
- 1. Introduction
- 2. Attributes of multiscale computational modeling for stem cell research
- 3. Bioinformatics approach in stem cell
- 4. Artificial intelligence based supervised and unsupervised modeling approach
- 5. Mechanistic modeling approach
- 6. Dynamical modeling approach
- 7. Spatiotemporal analysis
- 8. Comparing approaches & future perspectives of computational models for stem cell-based regenerative medicine therapies
- 9. Stem cell population modeling in wound healing
- 10. Insights into stem cell therapy for vision defects
- 11. Regeneration of pacemakers using stem cells
- 12. Interpretation of scRNA-seq for the usage of MSCs in regenerative engineering
- 13. Conclusion
- Chapter 32. Computational analysis in epithelial tissue regeneration
- 1. Introduction
- 2. Epithelial tissue
- 3. Physiology of epithelial tissue regeneration
- 4. Computational biology and bioinformatics
- 5. Computational analysis in epithelial tissue regeneration
- 6. Examples of computational models and tools in epithelial tissue regeneration
- 7. Conclusion
- Author index
- Index
- Edition: 1
- Published: January 12, 2024
- Imprint: Academic Press
- No. of pages: 750
- Language: English
- Paperback ISBN: 9780443132223
- eBook ISBN: 9780443132216
PR
Pawan Raghav
RK
Rajesh Kumar
AL
Anjali Lathwal
NS
Navneet Sharma
Dr. Navneet Sharma is an Assistant Professor at the Amity Institute of Pharmacy, Amity University, India. He has an M.Pharm, Ph.D and PGDRA, and his expertise lies in the realm of biomaterials and applied R & D, especially needs-based product development. He has taken pivotal roles as an investigator in three projects supported by DST-India. He has won several awards, including eight national and international awards. The most prestigious among them are SCO and Ministry of External Affairs, Government of India Covid-19 best innovation award 2020, and Department of Science and Technology, Young Scientist Award for the year 2018 and 2022. He has more than 40 publications including four books, five book chapters, 10 patents and 4 technologies successfully transferred to the industry.