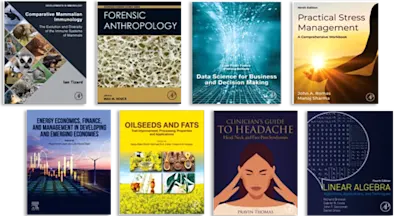
Computational Automation for Water Security
Enhancing Water Quality Management
- 1st Edition - February 27, 2025
- Imprint: Elsevier
- Editors: Ashutosh Kumar Dubey, Arun Lal Srivastav, Abhishek Kumar, Fausto Pedro Garcia Marquez, Dimitrios A Giannakoudakis
- Language: English
- Paperback ISBN:9 7 8 - 0 - 4 4 3 - 3 3 3 2 1 - 7
- eBook ISBN:9 7 8 - 0 - 4 4 3 - 3 3 3 2 2 - 4
Computational Automation for Water Security: Enhancing Water Quality Management is a comprehensive and insightful guide which explores the challenges posed by inefficient and ou… Read more
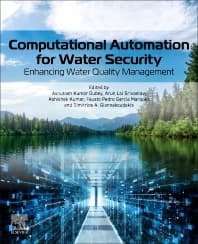
Purchase options
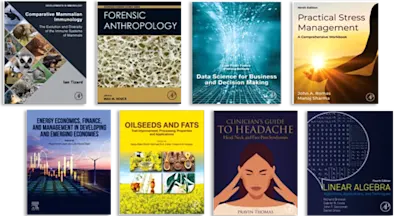
Institutional subscription on ScienceDirect
Request a sales quoteComputational Automation for Water Security: Enhancing Water Quality Management is a comprehensive and insightful guide which explores the challenges posed by inefficient and outdated practices, presenting innovative solutions to enhance decision-making, optimizing water treatment processes, and ultimately improving environmental outcomes. Through the coverage of advanced computational techniques, such as data analysis, machine learning, and optimization strategies, readers will gain a deep understanding of how computational automation can revolutionize decision-making. This book is an invaluable resource for professionals, researchers, and policymakers seeking to stay at the forefront of water quality management practices, harnessing the power of computational automation for a cleaner, healthier future.
- Offers a holistic understanding of the application of computational automation in water quality management
- Contains practical and unique updates to help learners how to apply computational techniques to address water quality challenges
- Provides a comprehensive and multidisciplinary perspective on water quality management
Water quality engineers and postgraduate students focusing on water quality management
- Title of Book
- Cover image
- Title page
- Table of Contents
- Copyright
- List of contributors
- About the editors
- Preface
- Chapter 1. Artificial intelligence and machine learning based water quality monitoring, prediction, and analysis: a comprehensive review
- Abstract
- 1.1 Introduction
- 1.2 Literature survey
- 1.3 Discussions
- 1.4 Conclusion and future work
- References
- Chapter 2. Advancements in water quality monitoring: leveraging machine learning and artificial intelligence for environmental management
- Abstract
- 2.1 Introduction
- 2.2 Literature survey
- 2.3 Discussions
- 2.4 Conclusion
- References
- Chapter 3. Revolutionizing water quality management the impact of machine learning and artificial intelligence
- Abstract
- 3.1 Introduction to machine learningand artificial intelligence in water quality monitoring
- 3.2 Overview of machine learning and artificial intelligence technologies
- 3.3 Revolutionizing water management
- 3.4 Automation and accuracy in monitoring processes
- 3.5 Future predictive capabilities of water quality
- 3.6 Enhancing efficiency and resource management
- 3.7 Applications of machine learning and artificial intelligence in automating water quality monitoring, analysis, and management
- 3.8 Discussion
- 3.9 Conclusion: toward global water security and sustainability
- References
- Chapter 4. Enhancing water quality management: the role of predictive modeling and IoT in monitoring, analysis, and intervention
- Abstract
- 4.1 Introduction
- 4.2 Developing a machine learning model for assessing and monitoring water quality parameters
- 4.3 Strategies for data acquisition: remote sensing, Internet of Things, and unmanned aerial vehicles
- 4.4 Applications of machine learning and artificial intelligence in water quality assessment
- 4.5 Real-life applications of artificial intelligence in water quality management
- 4.6 Discussion
- 4.7 Conclusions
- References
- Chapter 5. Enhancing water quality management through artificial intelligence and machine learning technologies
- Abstract
- 5.1 Introduction
- 5.2 Major artificial intelligence techniques used in water quality analysis, monitoring, and management
- 5.3 Prediction of various water quality parameters
- 5.4 Discussion
- 5.5 Conclusion
- References
- Chapter 6. Machine learning and artificial intelligence application in automotive water quality monitoring, analysis, and management
- Abstract
- Nomenclature
- 6.1 Introduction
- 6.2 Discussion section
- 6.3 Conclusion
- References
- Chapter 7. Integrating AI, machine learning, and nanotechnology: shaping the future of water quality management
- Abstract
- 7.1 Introduction
- 7.2 Fundamentals of water quality management
- 7.3 Role of AI in water quality management
- 7.4 Application of machine learning technology in water quality management
- 7.5 Nanotechnology for water quality management
- 7.6 Integration of AI, machine technology, and nanotechnology in water quality management
- 7.7 Discussion
- 7.8 Conclusion
- References
- Further reading
- Chapter 8. Empowering water security: the synergy of automation and IoT integration
- Abstract
- 8.1 Introduction
- 8.2 The synergy of automation and IoT
- 8.3 Enhanced infrastructure resilience
- 8.4 Future prospects and innovations
- 8.5 Conclusion and recommendations
- References
- Chapter 9. Cost-benefit analysis of automated water quality management: investment, savings, and strategic advantages
- Abstract
- 9.1 Introduction
- 9.2 Literature review
- 9.3 Initial investment and operational saving costs
- 9.4 Discussion
- 9.5 Conclusion
- References
- Further reading
- Chapter 10. Significance of automation in water treatment processes
- Abstract
- 10.1 Introduction
- 10.2 Various automation used in water treatment processes
- 10.3 Process automation used in wastewater treatment
- 10.4 Importance of automation in water treatment plants
- 10.5 Discussion
- 10.6 Conclusion
- References
- Chapter 11. Water sustainability: a review of advances in water quality management technologies
- Abstract
- 11.1 Introduction
- 11.2 Methodology
- 11.3 IoT and ML in WQM
- 11.4 Water sustainability, WQM, UN SDGs
- 11.5 Discussion
- 11.6 Conclusions
- References
- Chapter 12. Future directions in water quality management: integrating advanced technologies and sustainable practices
- Abstract
- 12.1 Introduction
- 12.2 Concept of water quality management and present technical scenario
- 12.3 Data analytics and predictive modeling
- 12.4 Decentralized water treatment systems and its significance
- 12.5 Emerging contaminant removal technique
- 12.6 Resource recovery and circular economy
- 12.7 Discussion
- 12.8 Policy and governance innovations
- 12.9 Conclusion
- References
- Chapter 13. Future trends and emerging technologies in water quality management
- Abstract
- 13.1 Introduction
- 13.2 Innovations and sustainable practices in water treatment technologies
- 13.3 Nanotechnology-based solutions
- 13.4 Membrane filtration advancements
- 13.5 Electrochemical water treatment methods
- 13.6 Nature-based solutions (constructed wetlands, biofilters)
- 13.7 Wastewater recycling and reuse
- 13.8 Low-energy and renewable energy technologies in water treatment
- 13.9 Emerging technologies in water quality monitoring
- 13.10 Real-time monitoring systems
- 13.11 Future trends in water quality management
- 13.12 Discussion
- 13.13 Conclusions
- References
- Chapter 14. Addressing complex challenges in water quality management: emerging technologies and sustainable strategies
- Abstract
- 14.1 Overview of water quality
- 14.2 The water-energy nexus
- 14.3 Current challenges in water quality management
- 14.4 Emerging trends in water quality management
- 14.5 Sustainable approaches in water quality management
- 14.6 Artificial intelligence and machine learning applications in water quality management
- 14.7 Advanced sensor technologies for monitoring water quality
- 14.8 Discussion
- 14.9 Conclusion
- 14.10 Future perspective (recommendation)
- References
- Chapter 15. Introduction to computational automation in water quality management
- Abstract
- Abbreviations
- 15.1 Introduction
- 15.2 Historical background
- 15.3 Role of computational automation in modern water quality management
- 15.4 Emergence of automation in water quality
- 15.5 Technologies in computational automation
- 15.6 Software and systems for water quality management
- 15.7 Automation techniques and methodologies
- 15.8 Case studies and industrial applications
- 15.9 Challenges and limitations
- 15.10 Future directions and innovations
- 15.11 Conclusion
- Acknowledgments
- References
- Chapter 16. Case studies: Successful implementation of automation for water quality assessment
- Abstract
- 16.1 Introduction
- 16.2 Literature review
- 16.3 Case study 1
- 16.4 Case study 2: future trends in automated water quality assessment
- 16.5 Case study 3: farm runoff and mitigation
- 16.6 Regional weather prediction models
- 16.7 Financial and environmental effects of the use of automation
- 16.8 Summary and improvements
- 16.9 Conclusion (which includes shortcomings from what was investigated and further ideas)
- 16.10 Conclusion
- References
- Chapter 17. Enhancing water security through automation: case studies and technical advancements in water quality management
- Abstract
- 17.1 Introduction
- 17.2 The rationale for adopting automation in water quality assessment
- 17.3 Fundamentals of computational automation
- 17.4 Definition and principles of computational automation
- 17.5 Role of computational methods in water quality assessment
- 17.6 Case study 1: automated sensor networks for real-time monitoring
- 17.7 Analysis of the effectiveness of real-time data collection in identifying pollution sources and assessing water quality trends
- 17.8 Case study 2: exploration of a case study employing machine learning algorithms to develop predictive models for water quality assessment
- 17.9 Considerations for scalability and integration with existing monitoring frameworks
- 17.10 Identification of challenges encountered in implementing automation for water quality assessment
- 17.11 Strategies for addressing challenges and maximizing the benefits of automation
- 17.12 Future directions and opportunities
- 17.13 Literature review
- 17.14 Key findings, interpretations, and implications
- 17.15 Conclusion
- References
- Chapter 18. Jal Jeevan Mission and role of smart technologies in achieving water security in rural India
- Abstract
- 18.1 Introduction
- 18.2 Global water status
- 18.3 Multifarious nexus of water security in India
- 18.4 Institutionalization of water security in changing climate in India
- 18.5 Importance of smart technology in attaining water security through water management
- 18.6 Critical discussion
- 18.7 Conclusion
- References
- Chapter 19. Computational automation for enhancing water security and quality management
- Abstract
- 19.1 Introduction
- 19.2 Technical challenges and integration with existing infrastructure
- 19.3 Financial challenges
- 19.4 Societal challenges
- 19.5 Ethical considerations in water automation
- 19.6 Addressing the challenges: technical solutions
- 19.7 Financial strategies for overcoming water automation challenges
- 19.8 Societal approaches to addressing challenges in water automation
- 19.9 Discussion
- 19.10 Future directions and recommendations
- 19.11 Conclusion
- References
- Chapter 20. Synergizing sustainability: integrating computational automation for water security and sustainable tourism
- Abstract
- 20.1 Introduction
- 20.2 Literature review
- 20.3 Integrating frameworks
- 20.4 New framework developed: integrated computational sustainability framework for water security and tourism
- 20.5 Discussion and conclusion
- References
- Chapter 21. The moral current: ethical dilemmas in water automation
- Abstract
- 21.1 Introduction
- 21.2 Literature review
- 21.3 Ethical considerations in AI-driven agriculture
- 21.4 Ethical conundrums in water automation with artificial intelligence
- 21.5 Challenges and future prospects
- 21.6 Discussion
- 21.7 Conclusion
- References
- Chapter 22. Applications of machine learning and artificial intelligence in automating water quality monitoring, analysis, and management
- Abstract
- 22.1 Introduction
- 22.2 Data acquisition and preprocessing
- 22.3 Machine learning for water quality analysis
- 22.4 AI-driven automation systems
- 22.5 Applications and case studies
- 22.6 Challenges and future directions
- 22.7 Conclusion
- References
- Index
- Edition: 1
- Published: February 27, 2025
- Imprint: Elsevier
- No. of pages: 498
- Language: English
- Paperback ISBN: 9780443333217
- eBook ISBN: 9780443333224
AD
Ashutosh Kumar Dubey
Ashutosh Kumar Dubey is an Associate Professor in the Department of Computer Science and Engineering at Chitkara University, Himachal Pradesh, India. He is also a Postdoctoral Fellow of the Ingenium Research Group Lab, Universidad
de Castilla-La Mancha, Ciudad Real, Spain.
Affiliations and expertise
Department of Computer Science and Engineering, Institute of Engineering and Technology, Chitkara University, IndiaAS
Arun Lal Srivastav
Dr. Arun Lal Srivastav is an Associate Professor in the Department of Applied Sciences at Chitkara University, Himachal Pradesh, India.
Affiliations and expertise
Chitkara University, Himachal Pradesh, Solan, IndiaAK
Abhishek Kumar
Dr. Abhishek Kumar is a professor and post-doctorate fellow in computer science at Ingenium Research Group, based at Universidad De Castilla-La Mancha in Spain. He has been teaching in academia for more than 8 years, and published more than 50 articles in reputed, peer reviewed national and international journals, books, and conferences. His research area includes artificial intelligence, image processing, computer vision, data mining, and machine learning.
Affiliations and expertise
Professor, Post-doctorate Fellow, Ingenium Research Group, Universidad De Castilla-La Mancha, SpainFG
Fausto Pedro Garcia Marquez
Fausto Pedro García Márquez works as a Professor and as Director of the Ingenium Research Group at the Universidad De Castilla-La Mancha, Spain. He is an Honorary Senior Research Fellow at Birmingham University, UK, and a Lecturer at the Postgraduate European Institute. He has published more than 150 papers and 31 books (Elsevier, Springer, Pearson, McGraw-Hill, Intech, IGI, Marcombo, AlfaOmega). He has been Principal Investigator in 4 European projects, 6 national projects, and more than 150 projects for universities, companies, and other institutions. His main interests are: Artificial Intelligence, Maintenance, Management, Renewable Energy, Transport, Advanced Analytics, and Data Science.
Affiliations and expertise
Professor, Universidad De Castilla-La Mancha, SpainDA
Dimitrios A Giannakoudakis
Dr. Dimitrios Giannakoudakis graduated as chemist from Aristotle University of Thessaloniki (AUTh) in Greece, where he obtained also two M.Sc. degrees (in Physical-chemistry & Electrochemistry and in New Educational Technologies). He received his PhD degree on “Nanotechnology and Materials Chemistry” from the City University of New York (CUNY) with full scholarship, in 02/2017. Then, he continued as postdoctoral-fellow and adjunct tutor at the City College of New York, AUTh, and the Institute of Physical Chemistry (IChF) of Polish Academy of Sciences. Afterwards, he served at IChF as adj. Assistant Professor and tutor of “Modern nano-Topics in Physical Chemistry”. Currently, he is Research Associate and tutor at AUTh.
He has co-authored more than 105 publications in leading peer-reviewed journals (avg. Impact Factor as 1st author above 11), with the articles to be cited more than 3000 times, one monograph by Springer, three edited books by Elsevier, more than 20 book chapters, and 3 invention patents.
For more details: www.DaGchem.com
Affiliations and expertise
Assistant Professor, Institute of Physical Chemistry of Polish Academy of Sciences, GreeceRead Computational Automation for Water Security on ScienceDirect