LIMITED OFFER
Save 50% on book bundles
Immediately download your ebook while waiting for your print delivery. No promo code needed.
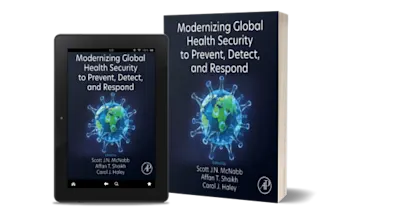
Computational and Network Modeling of Neuroimaging Data provides an authoritative and comprehensive overview of the many diverse modeling approaches that have been fruitfull… Read more
LIMITED OFFER
Immediately download your ebook while waiting for your print delivery. No promo code needed.
Computational and Network Modeling of Neuroimaging Data provides an authoritative and comprehensive overview of the many diverse modeling approaches that have been fruitfully applied to neuroimaging data. As neuroimaging is witnessing a massive increase in the quality and quantity of data being acquired, this book gives an accessible foundation to the field of computational neuroimaging, suitable for graduate students, academic researchers, and industry practitioners who are interested in adopting or applying model-based approaches in neuroimaging.
It is widely recognized that effective interpretation and extraction of information from complex data requires quantitative modeling. However, modeling the brain comes in many diverse forms, with different research communities tackling different brain systems, different spatial and temporal scales, and different aspects of brain structure and function. This book takes a critical step towards synthesizing and integrating across different modeling approaches.
KK