LIMITED OFFER
Save 50% on book bundles
Immediately download your ebook while waiting for your print delivery. No promo code needed.
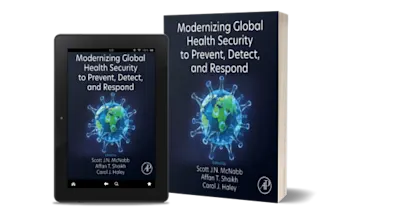
Computational and Data-Driven Chemistry Using Artificial Intelligence: Volume 1: Fundamentals, Methods and Applications highlights fundamental knowledge and current developme… Read more
LIMITED OFFER
Immediately download your ebook while waiting for your print delivery. No promo code needed.
Computational and Data-Driven Chemistry Using Artificial Intelligence: Volume 1: Fundamentals, Methods and Applications highlights fundamental knowledge and current developments in the field, giving readers insight into how these tools can be harnessed to enhance their own work. Offering the ability to process large or complex data-sets, compare molecular characteristics and behaviors, and help researchers design or identify new structures, Artificial Intelligence (AI) holds huge potential to revolutionize the future of chemistry. Volume 1 explores the fundamental knowledge and current methods being used to apply AI across a whole host of chemistry applications.
Drawing on the knowledge of its expert team of global contributors, the book offers fascinating insight into this rapidly developing field and serves as a great resource for all those interested in exploring the opportunities afforded by the intersection of chemistry and AI in their own work. Part 1 provides foundational information on AI in chemistry, with an introduction to the field and guidance on database usage and statistical analysis to help support newcomers to the field. Part 2 then goes on to discuss approaches currently used to address problems in broad areas such as computational and theoretical chemistry; materials, synthetic and medicinal chemistry; crystallography, analytical chemistry, and spectroscopy. Finally, potential future trends in the field are discussed.
1. Introduction to Computational and Data-Driven Chemistry Using AI
2. Goal-directed generation of new molecules by AI methods
3. Compounds based on structural database of X-ray crystallography
4. Approaches using AI in Medicinal Chemistry
5. Application of Machine learning algorithms for use in material chemistry
6. Predicting Conformers of Flexible Metal Complexes using Deep Neural Network
7. Predicting Activity and Activation Factor of Catalytic Reactions Using Machine Learning
8. Convolutional Neural Networks for the Design and Analysis of Non-Fullerene Acceptors
TA