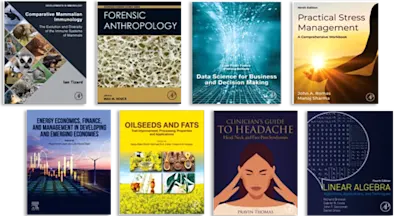
Cognitive Science, Computational Intelligence, and Data Analytics
Methods and Applications with Python
- 1st Edition - June 6, 2024
- Imprint: Morgan Kaufmann
- Authors: Vikas Khare, Sanjeet Kumar Dwivedi, Monica Bhatia
- Language: English
- Paperback ISBN:9 7 8 - 0 - 4 4 3 - 1 6 0 7 8 - 3
- eBook ISBN:9 7 8 - 0 - 4 4 3 - 1 6 0 7 9 - 0
Cognitive Science, Computational Intelligence, and Data Analytics: Methods and Applications with Python introduces readers to the foundational concepts of data analysis, cognit… Read more
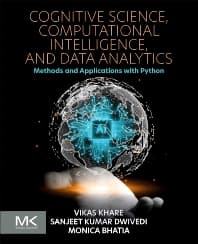
Purchase options
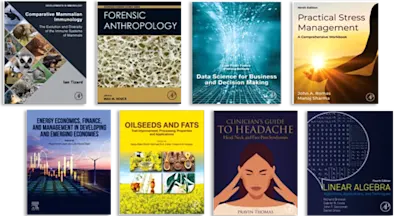
Institutional subscription on ScienceDirect
Request a sales quoteCognitive Science, Computational Intelligence, and Data Analytics: Methods and Applications with Python introduces readers to the foundational concepts of data analysis, cognitive science, and computational intelligence, including AI and Machine Learning. The book's focus is on fundamental ideas, procedures, and computational intelligence tools that can be applied to a wide range of data analysis approaches, with applications that include mathematical programming, evolutionary simulation, machine learning, and logic-based models. It offers readers the fundamental and practical aspects of cognitive science and data analysis, exploring data analytics in terms of description, evolution, and applicability in real-life problems.
The authors cover the history and evolution of cognitive analytics, methodological concerns in philosophy, syntax and semantics, understanding of generative linguistics, theory of memory and processing theory, structured and unstructured data, qualitative and quantitative data, measurement of variables, nominal, ordinals, intervals, and ratio scale data. The content in this book is tailored to the reader's needs in terms of both type and fundamentals, including coverage of multivariate analysis, CRISP methodology and SEMMA methodology. Each chapter provides practical, hands-on learning with real-world applications, including case studies and Python programs related to the key concepts being presented.
The authors cover the history and evolution of cognitive analytics, methodological concerns in philosophy, syntax and semantics, understanding of generative linguistics, theory of memory and processing theory, structured and unstructured data, qualitative and quantitative data, measurement of variables, nominal, ordinals, intervals, and ratio scale data. The content in this book is tailored to the reader's needs in terms of both type and fundamentals, including coverage of multivariate analysis, CRISP methodology and SEMMA methodology. Each chapter provides practical, hands-on learning with real-world applications, including case studies and Python programs related to the key concepts being presented.
- Demystifies the theory of data analytics using a step-by-step approach
- Covers the intersection of cognitive science, computational intelligence, and data analytics by providing examples and case studies with applied algorithms, mathematics, and Python programming code
- Introduces foundational data analytics techniques such as CRISP-DM, SEMMA, and Object Detection Models in the context of computational intelligence methods and tools
- Covers key concepts of multivariate and cognitive data analytics such as factor analytics, principal component analytics, linear regression analysis, logistic regression analysis, and value chain applications
Computer Science researchers, data science researchers, and data analysis researchers in academia and industry, Graduate students and senior undergraduate students in Computer Science, data science, and data analysis
- Cover image
- Title page
- Table of Contents
- Copyright
- Foreword
- Overview of the book
- Chapter 1. Foundation of analytics
- Abstract
- Abbreviations
- 1.1 Introduction
- 1.2 Concepts of analytics
- 1.3 Emerging areas of analytics
- 1.4 Value chain analysis
- 1.5 Conclusion
- 1.6 Case study
- 1.7 Exercise
- Further reading
- Chapter 2. Foundation of cognitive science
- Abstract
- Abbreviations
- 2.1 Introduction
- 2.2 Education
- 2.3 Philosophy
- 2.4 Artificial intelligence
- 2.5 Psychology
- 2.6 Neuroscience
- 2.7 Theoretical neuroscience
- 2.8 Linguistics
- 2.9 Anthropology
- 2.10 Cognitive science theories
- 2.11 Evaluation of cognitive science
- 2.12 Understanding brain and sensory motor information
- 2.13 Language and linguistic knowledge
- 2.14 Theory of information processing
- 2.15 Concept of short-term memory
- 2.16 Conclusion
- 2.17 Case study
- 2.18 Exercise
- 2.19 Assessment question
- Further reading
- Chapter 3. Data theory and taxonomy of data
- Abstract
- Abbreviations
- 3.1 Introduction
- 3.2 Data as a whole
- 3.3 Views of data
- 3.4 Measurement and scaling concepts
- 3.5 Various types of scale
- 3.6 Primary data analysis with Python
- 3.7 Conclusion
- 3.8 Case study
- 3.9 Exercise
- Further reading
- Chapter 4. Multivariate data analytics and cognitive analytics
- Abstract
- Abbreviations
- 4.1 Introduction
- 4.2 Factor analytics
- 4.3 Principal component analytics
- 4.4 Cluster analytics
- 4.5 Linear regression analysis
- 4.6 Logistic regression analysis
- 4.7 Application of analytics across value chain
- 4.8 Multivariate data analytics with Python
- 4.9 Conclusion
- 4.10 Case study
- 4.11 Exercise
- Further reading
- Chapter 5. Artificial intelligence and machine learning application in data analysis
- Abstract
- Abbreviations
- 5.1 Introduction
- 5.2 Fundamentals of artificial intelligence
- 5.3 Spectrum of artificial intelligence
- 5.4 Knowledge representation of artificial intelligence
- 5.5 Constraint satisfaction problem
- 5.6 Cognitive analysis with artificial intelligence
- 5.7 Conclusion
- 5.8 Case study
- 5.9 Exercise
- Further reading
- Chapter 6. Data analysis applications and methodology
- Abstract
- Abbreviations
- 6.1 Introduction
- 6.2 CRISP-DM methodology
- 6.3 SEMMA methodology
- 6.4 Real life work with artificial intelligence, machine learning, and deep learning
- 6.5 Object detection models
- 6.6 Conclusion
- 6.7 Case study
- 6.8 Exercise
- Further reading
- Glossary
- Index
- Edition: 1
- Published: June 6, 2024
- Imprint: Morgan Kaufmann
- No. of pages: 332
- Language: English
- Paperback ISBN: 9780443160783
- eBook ISBN: 9780443160790
VK
Vikas Khare
Vikas Khare, PhD, is an associate professor in the School of Technology, Management and Engineering, NMIMS, India, and is a certified energy manager in the Bureau of Energy Efficiency in India. He is a fellow member of Scholars Academic and Scientific Society, India. Dr. Khare’s main research interests are renewable energy systems, data analysis, artificial intelligence, optimization techniques, game theory, and big data.
Affiliations and expertise
Associate Professor, School of Technology, Management and Engineering NMIMS, Indore, India, Certified Energy Manager, Bureau of Energy Efficiency IndiaSD
Sanjeet Kumar Dwivedi
Sanjeet Kumar Dwivedi, PhD, is an adjunct professor at Curtin University, Perth, Australia and is working as a scrum master and senior R&D engineer in control engineering, application, and motor control for Danfoss Power Electronics, Graasten, Denmark since 2008, where he is conducting research on new control techniques in power electronics and motor drives. He has authored more than 40 technical papers and holds 3 international patents.
Affiliations and expertise
Scrum Master and Senior R&D Engineer, Danfoss Power Electronics, Adjunct Professor, Curtin University, AustraliaMB
Monica Bhatia
Monica Bhatia, PhD, is a faculty member at the School of Business Management, NMIMS, India. She holds an MBA and a PhD and is certified by NET, NCFM, and IRDA. Dr. Bhatia has extensive professional experience in training and business development within
both corporate and educational sectors, covering a wide range of areas such as renewable energy, operational management, data analysis, cognitive science, career development, marketing management, communication skills, interpersonal relations, and workplace professionalism. She has conducted life skills training for students and trainers from various nationalities and with diverse accents, and has been involved in consulting projects on e-commerce, product strategy, marketing strategy, and business processes.
Affiliations and expertise
School of Business Management, NMIMS, Indore, IndiaRead Cognitive Science, Computational Intelligence, and Data Analytics on ScienceDirect