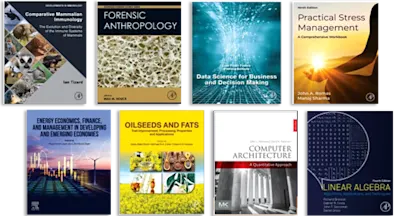
Cheminformatics, QSAR and Machine Learning Applications for Novel Drug Development
- 1st Edition - May 25, 2023
- Imprint: Academic Press
- Editor: Kunal Roy
- Language: English
- Paperback ISBN:9 7 8 - 0 - 4 4 3 - 1 8 6 3 8 - 7
- eBook ISBN:9 7 8 - 0 - 4 4 3 - 1 8 6 3 9 - 4
Cheminformatics, QSAR and Machine Learning Applications for Novel Drug Development aims at showcasing different structure-based, ligand-based, and machine learning tools currently… Read more
Purchase options
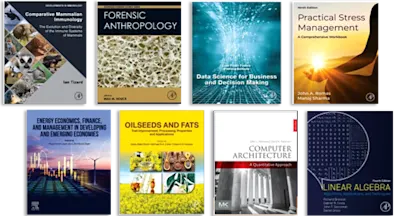
Cheminformatics, QSAR and Machine Learning Applications for Novel Drug Development aims at showcasing different structure-based, ligand-based, and machine learning tools currently used in drug design. It also highlights special topics of computational drug design together with the available tools and databases. The integrated presentation of chemometrics, cheminformatics, and machine learning methods under is one of the strengths of the book.
The first part of the content is devoted to establishing the foundations of the area. Here recent trends in computational modeling of drugs are presented. Other topics present in this part include QSAR in medicinal chemistry, structure-based methods, chemoinformatics and chemometric approaches, and machine learning methods in drug design. The second part focuses on methods and case studies including molecular descriptors, molecular similarity, structure-based based screening, homology modeling in protein structure predictions, molecular docking, stability of drug receptor interactions, deep learning and support vector machine in drug design. The third part of the book is dedicated to special topics, including dedicated chapters on topics ranging from de design of green pharmaceuticals to computational toxicology. The final part is dedicated to present the available tools and databases, including QSAR databases, free tools and databases in ligand and structure-based drug design, and machine learning resources for drug design. The final chapters discuss different web servers used for identification of various drug candidates.
- Presents chemometrics, cheminformatics and machine learning methods under a single reference
- Showcases the different structure-based, ligand-based and machine learning tools currently used in drug design
- Highlights special topics of computational drug design and available tools and databases
Section I: Introduction
1. Quantitative structure-activity relationships (QSARs) in medicinal chemistry
Kunal Roy and Mainak Chatterjee
2. Computer-aided Drug Design – An overview
Athina Geronikaki, Gurudutt Dubey, Anthi Petrou and Sivapriya Kirubakaran
3. Structure-based virtual screening in Drug Discovery
M R. Yadav, Prashant R. Murumkar, Rasana Yadav and Karan Joshi
4. The impact of Artificial Intelligence methods on drug design
Giuseppina Gini
Section 2. Methods and Case studies
5. Graph Machine Learning in Drug Discovery
Mohit Pandey, Atia Hamidizadeh, Mariia Radaeva, Michael Fernandez, Martin Ester and Artem Cherkasov
6. Support Vector Machine in Drug Design
Jose Isagani B. Janairo
7. Understanding protein-ligand interactions using state-of-the-art computer simulation methods
Elvis A. F. Martis, Manas Mahale, Aishwarya Chaudhari and Evans C. Coutinho
8. Structure-based methods in drug design
Lalitha Guruprasad, Priyanka Andola, Adrija Banerjee, Durgam Laxman and Gatta K.R.S. Naresh
9. Structure-based virtual screening
Shweta Singh Chauhan, Tanya Jamal, Anurag Singh, Ashish Sehrawat and Ramakrishnan Parthasarathi
10. Deep learning in drug design
Roi Naveiro, María Jimena Martínez, Axel J. Soto, Ignacio Ponzoni, David Ríos-Insua, and Nuria E. Campillo
11. Computational methods in the analysis of viral-host interactions
Olga A. Tarasova, Sergey M. Ivanov, Nadezhda Yu Biziukova, Shuanat Sh Kabieva and Vladimir V. Poroikov
12. Chemical space and Molecular Descriptors for QSAR studies
Viviana Consonni, Davide Ballabio and Roberto Todeschini
13. Machine learning methods in drug design
Gabriel Corrêa Veríssimo, Jadson de Castro Gertrudes and Vinícius Gonçalves Maltarollo
14. Deep learning methodologies in drug design
Haralambos Sarimveis, Chrysoula Gousiadou, Philip Doganis, Pantelis Karatzas, Iason Sotiropoulos and Periklis Tsiros
15. Molecular dynamics in predicting stability of drug receptor interactions
Dr. B Jayaram and Devendra Prajapat
Section 3. Special topics
16. Towards models for bioaccumulation suitable for the pharmaceutical domain
Davide Luciani, Erika Colombo, Anna Lombardo and Emilio Benfenati
17. Machine Learning as a Modeling Approach for the Account of Nonlinear Information in MIA-QSAR Applications: A Case Study with SVM Applied to Antimalarial (Aza)aurones
Joyce K. Daré, Adriana C. de Faria, Ingrid V. Pereira and Matheus P. Freitas
18. Deep Learning using molecular image of chemical structure
Yasunari Matsuzaka and Yoshihiro Uesawa
19. Recent Advances in Deep Learning Enabled Approaches for Identification of Molecules of Therapeutics Relevance
Kushagra Kashyap and Mohammad Imran Siddiqi
20. Computational toxicology of pharmaceuticals
Gulcin Tugcu, Hande Sipahi, Mohammad Charehsaz, Ahmet Aydın, and Melek Türker Saçan
21. Ecotoxicological QSAR modelling of pharmaceuticals
Elifcan Çalışkan, Gulcin Tugcu, Serli Önlü, and Melek Türker Saçan
22. Computational modelling of drugs for neglected diseases
Pablo Duchowicz, Silvina Fioressi and Daniel E. Bacelo
23. Modelling ADMET properties based on Biomimetic Chromatographic Data
Theodosia Vallianatou, Fotios Tsopelas and Anna Tsantili-Kakoulidou
24. A systematic chemoinformatic analysis of chemical space, scaffolds and antimicrobial activity of LpxC inhibitors
Sapna Swarup, Sonali Chhabra and Raman Parkesh
Section 4. Tools and databases
25. Tools and Software for Computer Aided Drug Design and Discovery
Siyun Yang, Supratik Kar and Jerzy Leszczynski
26. Machine learning resources for drug design
Nicola Gambacorta, Daniela Trisciuzzi, Fulvio Ciriaco, Fabrizio Mastrolorito, Maria Vittoria Togo, Anna Rita Tondo, Cosimo Damiano Altomare, Nicola Amoroso and Orazio Nicolotti
27. Building Bioinformatics Web Applications with Streamlit
Chanin Nantasenamat, Avratanu Biswas, J. M. Nápoles-Duarte, Mitchell I. Parker and Roland L. Dunbrack Jr
28. Free tools and databases in ligand and structure-based drug design
Pratibha Chaurasia, Anasuya Bhargav and Srinivasan Ramachandran
- Edition: 1
- Published: May 25, 2023
- Imprint: Academic Press
- Language: English
KR