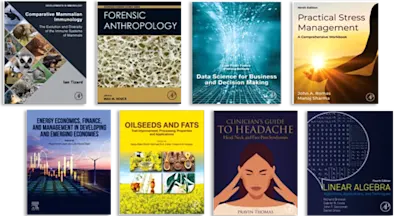
Brain Seizure Detection and Classification Using EEG Signals
- 1st Edition - September 9, 2021
- Imprint: Academic Press
- Authors: Varsha K. Harpale, Vinayak Bairagi
- Language: English
- Paperback ISBN:9 7 8 - 0 - 3 2 3 - 9 1 1 2 0 - 7
- eBook ISBN:9 7 8 - 0 - 3 2 3 - 9 1 1 2 1 - 4
Brain Seizure Detection and Classification Using Electroencephalographic Signals presents EEG signal processing and analysis with high performance feature extraction. The book cove… Read more
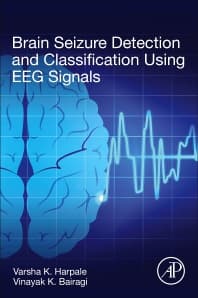
Purchase options
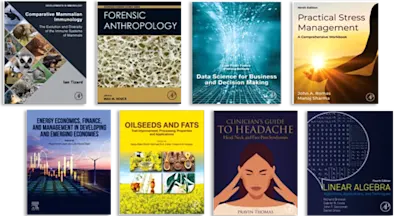
Institutional subscription on ScienceDirect
Request a sales quoteBrain Seizure Detection and Classification Using Electroencephalographic Signals presents EEG signal processing and analysis with high performance feature extraction. The book covers the feature selection method based on One-way ANOVA, along with high performance machine learning classifiers for the classification of EEG signals in normal and epileptic EEG signals. In addition, the authors also present new methods of feature extraction, including Singular Spectrum-Empirical Wavelet Transform (SSEWT) for improved classification of seizures in significant seizure-types, specifically epileptic and Non-Epileptic Seizures (NES). The performance of the system is compared with existing methods of feature extraction using Wavelet Transform (WT) and Empirical Wavelet Transform (EWT).
The book's objective is to analyze the EEG signals to observe abnormalities of brain activities called epileptic seizure. Seizure is a neurological disorder in which too many neurons are excited at the same time and are triggered by brain injury or by chemical imbalance.
- Presents EEG signal processing and analysis concepts with high performance feature extraction
- Discusses recent trends in seizure detection, prediction and classification methodologies
- Helps classify epileptic and non-epileptic seizures where misdiagnosis may lead to the unnecessary use of antiepileptic medication
- Provides new guidance and technical discussions on feature-extraction methods and feature selection methods based on One-way ANOVA, along with high performance machine learning classifiers for classification of EEG signals in normal and epileptic EEG signals, and new methods of feature extraction developed by the authors, including Singular Spectrum-Empirical Wavelet
- Cover image
- Title page
- Table of Contents
- Copyright
- Dedication
- Preface
- Acknowledgments
- Chapter 1: Introduction
- Abstract
- 1.1. Introduction
- 1.2. Methods of brain analysis
- 1.3. Recent trends in EEG analysis for feature extraction
- 1.4. Trends in artifact removal and FPGA architecture designs
- 1.5. Seizure detection techniques
- 1.6. Classification of epileptic and nonepileptic seizures
- 1.7. Seizure prediction techniques
- 1.8. Risk analysis of seizures
- 1.9. Summary of seizure detection, prediction, and classification
- References
- Chapter 2: Concepts of EEG analysis and seizures
- Abstract
- 2.1. EEG analysis and seizure detection methodology
- 2.2. Brain signal behavior, measurement, and recording
- 2.3. EEG processing and mathematical model
- 2.4. Artifacts observed in EEG signals
- 2.5. Epilepsy
- 2.6. Summary
- References
- Chapter 3: Seizure detection methods and analysis
- Abstract
- 3.1. EEG feature extraction
- 3.2. Improved seizure detection methods
- 3.3. Feature extraction for seizure detection
- 3.4. Classification methods
- 3.5. Hardware architecture for seizure detection
- 3.6. Result and performance analysis of seizure detection
- 3.7. Summary
- References
- Chapter 4: Pattern adapted wavelet-based seizure prediction
- Abstract
- 4.1. Recent trends in epileptic seizure detection using fuzzy classifiers
- 4.2. Dataset
- 4.3. Feature extraction
- 4.4. Classification model for preseizure, seizure, and normal states
- 4.5. Feature extraction for preseizure identification
- 4.6. Performance evaluation
- 4.7. Summary
- References
- Chapter 5: Effective method for epileptic and nonepileptic seizure classification
- Abstract
- 5.1. Introduction
- 5.2. Dataset used for experimentation
- 5.3. Seizure classification
- 5.4. Classification using machine learning classifiers
- 5.5. Results and discussion
- 5.6. Risk analysis model
- 5.7. Summary
- References
- Chapter 6: Concluding remarks and future scope
- Abstract
- 6.1. Seizure detection results
- 6.2. Seizure prediction results
- 6.3. Seizure classification results
- 6.4. Summarized observations
- 6.5. Conclusions
- 6.6. Future scope
- References
- Index
- Edition: 1
- Published: September 9, 2021
- Imprint: Academic Press
- No. of pages: 176
- Language: English
- Paperback ISBN: 9780323911207
- eBook ISBN: 9780323911214
VH
Varsha K. Harpale
VB