AI & BIG DATA
Shaping today's innovations
Save up to 25% on AI & Big Data books, eBooks & Journals
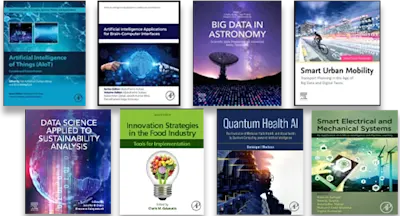
Big Data in Psychiatry and Neurology provides an up-to-date overview of achievements in the field of big data in Psychiatry and Medicine, including applications of big data meth… Read more
AI & BIG DATA
Save up to 25% on AI & Big Data books, eBooks & Journals
Big Data in Psychiatry and Neurology provides an up-to-date overview of achievements in the field of big data in Psychiatry and Medicine, including applications of big data methods to aging disorders (e.g., Alzheimer’s disease and Parkinson’s disease), mood disorders (e.g., major depressive disorder), and drug addiction. This book will help researchers, students and clinicians implement new methods for collecting big datasets from various patient populations. Further, it will demonstrate how to use several algorithms and machine learning methods to analyze big datasets, thus providing individualized treatment for psychiatric and neurological patients.
As big data analytics is gaining traction in psychiatric research, it is an essential component in providing predictive models for both clinical practice and public health systems. As compared with traditional statistical methods that provide primarily average group-level results, big data analytics allows predictions and stratification of clinical outcomes at an individual subject level.
Researchers and students in Psychiatry and Neurology designing protocols; Clinicians involved in clinical trials
1. Best practices for supervised machine learning when examining biomarkers in clinical populations
Benjamin G. Schultz, Zaher Joukhadar, Usha Nattala, Maria del Mar Quiroga, Francesca Bolk, and Adam P. Vogel
2. Big data in personalized healthcare
Lidong Wang and Cheryl Alexander
3. Longitudinal data analysis: The multiple indicators growth curve model approach
Thierno M.O. Diallo and Ahmed A. Moustafa
4. Challenges and solutions for big data in personalized healthcare
Tim Hulsen
5. Data linkages in epidemiology
Sinead Moylett
6. Neutrosophic rule-based classification system and its medical applications
Sameh H. Basha, Areeg Abdalla, and Aboul Ella Hassanien
7. From complex to neural networks
Nicola Amoroso and Loredana Bellantuono
8. The use of Big Data in psychiatry—The role of administrative databases
Manuel Goncalves-Pinho and Alberto Freitas
9. Predicting the emergence of novel psychoactive substances with big data
Robert Todd Perdue and James Hawdon
10. Hippocampus segmentation in MR images: Multiatlas methods and deep learning methods
Hancan Zhu, Shuai Wang, Liangqiong Qu, and Dinggang Shen
11. A scalable medication intake monitoring system
Diane Myung-Kyung Woodbridge and Kevin Bengtson Wong
12. Evaluating cascade prediction via different embedding techniques for disease mitigation
Abhinav Choudhury, Shubham Shakya, Shruti Kaushik, and Varun Dutt
13. A two-stage classification framework for epileptic seizure prediction using EEG wavelet-based features
Sahar Elgohary, Mahmoud I. Khalil, and Seif Eldawlatly
14. Visual neuroscience in the age of big data and artificial intelligence
Kohitij Kar
15. Application of big data and artificial intelligence approaches in diagnosis and treatment of neuropsychiatric diseases
Qiurong Song, Tianhui Huang, Xinyue Wang, Jingxiao Niu, Wang Zhao, Haiqing Xu, and Long Lu
16. Leveraging big data to augment evidence-informed precise public health response
G.V. Asokan and Mohammed Yousif Abbas Mohammed
17. How big data analytics is changing the face of precision medicine in women‘s health
Maryam Panahiazar, Maryam Karimzadehgan, Roohallah Alizadehsani, Dexter Hadley, and Ramin E. Beygui
AM