Limited Offer
Save 50% on book bundles
Immediately download your ebook while waiting for your print delivery. No promo code needed.
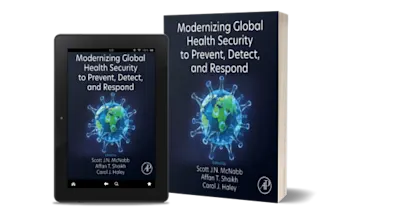
Big Data Application in Power Systems, Second Edition presents a thorough update of the previous volume, providing readers with step-by-step guidance in big data analytics… Read more
Limited Offer
Big Data Application in Power Systems, Second Edition presents a thorough update of the previous volume, providing readers with step-by-step guidance in big data analytics utilization for power system diagnostics, operation, and control. Divided into three parts, this book begins by breaking down the big picture for electric utilities before zooming in to examine theoretical problems and solutions in detail. Finally, the third section provides case studies and applications, demonstrating solution troubleshooting and design from a variety of perspectives and for a range of technologies.
Bringing back a team of global experts and drawing on fresh, emerging perspectives, this book provides cutting-edge advice for meeting today’s challenges in this rapidly accelerating area of power engineering.
Readers will develop new strategies and techniques for leveraging data towards real-world outcomes.
Researchers, graduate students, professors, and lecturers in electricity networks and smart grids. Scientists and engineers, data analysis experts and software developers who are working on electricity networks and advanced technologies for smart grids
RA
YZ