LIMITED OFFER
Save 50% on book bundles
Immediately download your ebook while waiting for your print delivery. No promo code needed.
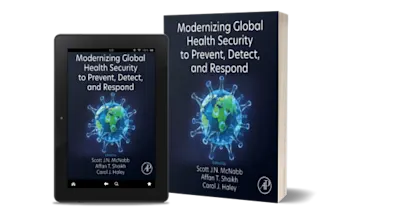
Between the Lines of Genetic Code lays out methodologies and tools for the measurement and evaluation of gene-gene and gene-environment studies and gives perspective on the futur… Read more
LIMITED OFFER
Immediately download your ebook while waiting for your print delivery. No promo code needed.
Between the Lines of Genetic Code lays out methodologies and tools for the measurement and evaluation of gene-gene and gene-environment studies and gives perspective on the future of this discipline. The book begins by defining terms for interaction studies, describing methodologies, and critically assessing the viability of current study designs and the possibilities for integrating designs. It then provides recent applications data with case studies in rheumatoid arthritis, multiple sclerosis, myositis and other complex human diseases. Last, it examines current studies and directions for future applications in patient care.
Recent multivariate studies show that gene-gene and gene-environment interactions can explain significant variances in inheritance that have previously been undetectable in univariate analysis. These links among genes and between genes and their environments during the development of diseases may serve as important hints for understanding pathogenic mechanisms and for developing new tools for prognosis, diagnosis, and treatment of various diseases.
Research geneticists; molecular and cell biologists; biologists, bioinformaticians, and biostatisticians with interests in human disease
Acknowledgments
List of Contributors
Introduction
Interaction May be Confused with Many Different Things
Genetics in Human Diseases
Complex Human Diseases
Genome-Wide Association Studies as a Source of Information
References
Part One: Methodology
Chapter One. Terminology and Definitions for Interaction Studies
1.1 Introduction
1.2 Regression Models
1.3 The Linear Regression Model
1.4 Assumptions of Linear Regression Models
1.5 Estimation
1.6 Interaction on the Linear Scale
1.7 Logistic Regression Models
1.8 Other Nonlinear Regression Models
1.9 The Hazard Function and the Survival Function
1.10 Hazard Ratio
1.11 Assumptions
1.12 Interaction on the Logistic Scale
1.13 Estimating Multiplicative Interaction
1.14 Estimating Additive Interaction
1.15 Genetic Models
1.16 Summary
References
Chapter Two. Reducing Dimensionality in the Search for Gene–Gene Interactions
2.1 Introduction
2.2 Challenges in Searching for Gene–Gene Interactions
2.3 Data Mining Approaches for Gene–Gene Interactions
2.4 Example of a Data Mining Method: MDR
2.5 Strategies to Improve Power of Data Mining Methods
2.6 Computational Optimizations
2.7 Future of Gene–Gene Interaction Modeling
2.8 Conclusions
References
Chapter Three. Study Design for Interaction Analyses
3.1 Introduction
3.2 Study Base
3.3 Cohort Studies
3.4 Case-Control Studies
3.5 Systematic and Random Errors
3.6 Example of Gene–Environment Interaction
3.7 Summary
References
Chapter Four. High-Throughput Genetic Interaction Study
4.1 Introduction
4.2 Gene–Gene Interactions
4.3 Gene–Environment Interactions
4.4 Novel Statistic for Genome-Wide Interaction Analysis
4.5 Conclusions
References
Appendix 4.1 The Algorithm of the Forest-Based Haplotype Approach
Appendix 4.2 The FEPI-MB algorithm
Appendix 4.3 The bNEAT Algorithm
Appendix 4.4 The GEIRA Algorithm
Part 1. Conclusions
Reference
Part Two: Experimental Data
Chapter Five. Gene–Gene and Gene–Environment Interaction in Rheumatoid Arthritis
5.1 Introduction
5.2 Phenotypic Heterogeneity of Rheumatoid Arthritis: ACPAs
5.3 Genetic Risk Factors for Rheumatoid Arthritis
5.4 Nongenetic Factors in Risk for Rheumatoid Arthritis (Table 5.2)
5.5 Gene–Environment and Gene–Gene Interactions in Rheumatoid Arthritis (Table 5.3)
Conclusions
References
Chapter Six. Genetic and Environmental Risk Factors for Multiple Sclerosis—A Role for Interaction Analysis
6.1 Genetics of Multiple Sclerosis
6.2 Environmental Risk Factors for Multiple Sclerosis
6.3 Study of Interactions in Multiple Sclerosis
6.4 Gene–Gene Interactions
6.5 Gene–Environment Interactions
6.6 Conclusions: What Have We Learnt?
References
Chapter Seven. Gene–Gene and Gene–Environment Interactions in Defining Risk and Spectrum of Phenotypes in Idiopathic Inflammatory Myopathies
7.1 Clinical Introduction
7.2 Early HLA Results in Idiopathic Inflammatory Myopathies
7.3 HLA-Related Differences in PM/DM
7.4 The Association Between HLA Genes, Class II Haplotypes, and MSAs/MAAs
7.5 Contribution of HLA-DRB1 Gene Dose to Disease Phenotype and Severity in IBM
7.6 Remarkable Lessons from Statin-Induced Myositis
7.7 Cancer-Associated Myositis (CAM), HLA, and Anti-155/140 Autoantibodies
7.8 Relationship Between Smoking, HLA-DRB1*03, and Anti-Jo-1 in IIM
7.9 Possible Pathogenic Role of HLA and Autoantibodies
References
Part 2. Conclusions
References
Part Three: Future Avenues
Chapter Eight. Functional Studies of Gene–Gene Interaction of Autoimmune Diseases
8.1 Introduction
8.2 Attribution of Genetic Variation to Gene Function
8.3 Examples of Experimental Studies of Gene–Gene Interaction in Autoimmune Diseases
8.4 Conclusions
References
Chapter Nine. Genetic Vectors Approach in a Study of Fine Structure of Interaction Between Risk Haplotype of HTR2A and HLA-DRB1 Shared Epitope Alleles in Rheumatoid Arthritis
9.1 Introduction
9.2 Methods
9.3 Results
9.4 Discussion
References
Appendix Standard Correlations and Statistical Interaction
Chapter Ten. Network Biology Empowering Detection and Understanding of Interactions Between Genetic Factors in Development of Complex Phenotypes
10.1 Rise of Big Data, Computing, and Prediction
10.2 Using Genetic Variants as Independent Features is Not Sufficient for Realizing P4 Medicine
10.3 Network Biology—A Framework for Detecting and Interpreting Genetic Interactions
10.4 Inferring Genetic Interactions or Edges From Data is a Special Case of a Feature Selection Problem
10.5 Network Biology—A Framework for Detecting Genetic Interactions
Acknowledgments
References
Part 3. Conclusions
Index