ROBOTICS & AUTOMATION
Empowering Progress
Up to 25% off Essentials Robotics and Automation titles
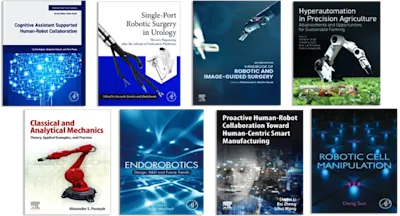
Bayesian Data Analysis in Ecology Using Linear Models with R, BUGS, and STAN examines the Bayesian and frequentist methods of conducting data analyses. The book provides the the… Read more
ROBOTICS & AUTOMATION
Up to 25% off Essentials Robotics and Automation titles
Bayesian Data Analysis in Ecology Using Linear Models with R, BUGS, and STAN examines the Bayesian and frequentist methods of conducting data analyses. The book provides the theoretical background in an easy-to-understand approach, encouraging readers to examine the processes that generated their data. Including discussions of model selection, model checking, and multi-model inference, the book also uses effect plots that allow a natural interpretation of data.
Bayesian Data Analysis in Ecology Using Linear Models with R, BUGS, and STAN introduces Bayesian software, using R for the simple modes, and flexible Bayesian software (BUGS and Stan) for the more complicated ones. Guiding the ready from easy toward more complex (real) data analyses ina step-by-step manner, the book presents problems and solutions—including all R codes—that are most often applicable to other data and questions, making it an invaluable resource for analyzing a variety of data types.
Graduate students and professionals in ecology, biogeography, and biology
FK
TR
Sv
JG
BA
PK