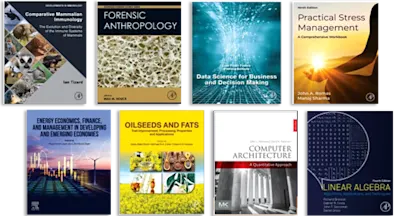
Augmenting Neurological Disorder Prediction and Rehabilitation Using Artificial Intelligence
- 1st Edition - February 24, 2022
- Imprint: Academic Press
- Editors: Anitha S. Pillai, Bindu Menon
- Language: English
- Paperback ISBN:9 7 8 - 0 - 3 2 3 - 9 0 0 3 7 - 9
- eBook ISBN:9 7 8 - 0 - 3 2 3 - 8 8 6 2 6 - 0
Augmenting Neurological Disorder Prediction and Rehabilitation Using Artificial Intelligence focuses on how the neurosciences can benefit from advances in AI, especially in areas… Read more
Purchase options
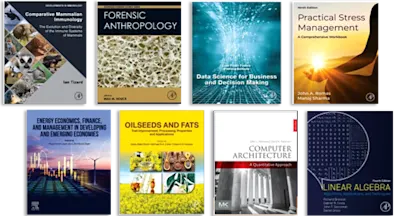
Augmenting Neurological Disorder Prediction and Rehabilitation Using Artificial Intelligence focuses on how the neurosciences can benefit from advances in AI, especially in areas such as medical image analysis for the improved diagnosis of Alzheimer’s disease, early detection of acute neurologic events, prediction of stroke, medical image segmentation for quantitative evaluation of neuroanatomy and vasculature, diagnosis of Alzheimer’s Disease, autism spectrum disorder, and other key neurological disorders. Chapters also focus on how AI can help in predicting stroke recovery, and the use of Machine Learning and AI in personalizing stroke rehabilitation therapy.
Other sections delve into Epilepsy and the use of Machine Learning techniques to detect epileptogenic lesions on MRIs and how to understand neural networks.
- Provides readers with an understanding on the key applications of artificial intelligence and machine learning in the diagnosis and treatment of the most important neurological disorders
- Integrates recent advancements of artificial intelligence and machine learning to the evaluation of large amounts of clinical data for the early detection of disorders such as Alzheimer’s Disease, autism spectrum disorder, Multiple Sclerosis, headache disorder, Epilepsy, and stroke
- Provides readers with illustrative examples of how artificial intelligence can be applied to outcome prediction, neurorehabilitation and clinical exams, including a wide range of case studies in predicting and classifying neurological disorders
2. Deep Learning for Non-Invasive Management of Brain Tumors
3. AI in Parkinson’s disease – symptoms identification and monitoring
4. Alzheimer’s Disease Detection using Artificial Intelligence
5. Intelligent Computer Systems for Multiple Sclerosis Diagnosis
6. Current and future applications of artificial intelligence in Multiple Sclerosis
7. Artificial Intelligence Assisted Headache Classification Methods
8. Deep Learning for Reliable Detection of Epileptogenic Lesions
9. AI in Neurosciences – Are we really there?
10. AI in the management of neurological disorders: Its prevalence and prominence
11. Graphical assessment of internal structure of some Parkinson disease data - A case study
12. Applications of Artificial Intelligence to Neurological Disorders: A Review of Current Technologies and Open Problems
13. Developing a Chatbot/Intelligent system for Neurological diagnosis and management
14. Artificial Intelligence (AI) in the diagnosis and management of Acute Ischemic Stroke (AIS)
- Edition: 1
- Published: February 24, 2022
- Imprint: Academic Press
- Language: English
AP
Anitha S. Pillai
BM