ROBOTICS & AUTOMATION
Empowering Progress
Up to 25% off Essentials Robotics and Automation titles
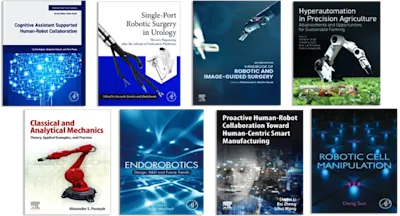
Artificial Intelligence in Urologic Malignancies describes current artificial intelligence technology, with an emphasis on prostate cancer applications. The book provides guidance… Read more
ROBOTICS & AUTOMATION
Up to 25% off Essentials Robotics and Automation titles
Artificial Intelligence in Urologic Malignancies describes current artificial intelligence technology, with an emphasis on prostate cancer applications. The book provides guidance on how artificial intelligence can improve therapeutics, how the power of artificial intelligence integrated with current standard therapy and research can enhance decision-making, and proposes future directions on how to integrate artificial intelligence within clinical applications. This is the perfect reference for scientists and researchers interested in the basic translational research opportunities such as drug discovery, pharmacogenetics, and experimental therapeutics, as well as clinicians interested in how AI applications are integrated with applications.
Researchers of prostate cancer, urologists and oncologists. Bioinformatics specialists, software engineers, data scientists
HA