Artificial Intelligence in the Age of Neural Networks and Brain Computing
- 2nd Edition - October 10, 2023
- Editors: Robert Kozma, Cesare Alippi, Yoonsuck Choe, Francesco Carlo Morabito
- Language: English
- Paperback ISBN:9 7 8 - 0 - 3 2 3 - 9 6 1 0 4 - 2
- eBook ISBN:9 7 8 - 0 - 3 2 3 - 9 5 8 1 6 - 5
Artificial Intelligence in the Age of Neural Networks and Brain Computing, Second Edition demonstrates that present disruptive implications and applications of AI is a developme… Read more
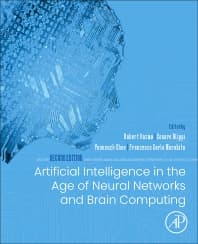
Purchase options
Institutional subscription on ScienceDirect
Request a sales quoteArtificial Intelligence in the Age of Neural Networks and Brain Computing, Second Edition demonstrates that present disruptive implications and applications of AI is a development of the unique attributes of neural networks, mainly machine learning, distributed architectures, massive parallel processing, black-box inference, intrinsic nonlinearity, and smart autonomous search engines. The book covers the major basic ideas of "brain-like computing" behind AI, provides a framework to deep learning, and launches novel and intriguing paradigms as possible future alternatives.
The present success of AI-based commercial products proposed by top industry leaders, such as Google, IBM, Microsoft, Intel, and Amazon, can be interpreted using the perspective presented in this book by viewing the co-existence of a successful synergism among what is referred to as computational intelligence, natural intelligence, brain computing, and neural engineering. The new edition has been updated to include major new advances in the field, including many new chapters.
- Developed from the 30th anniversary of the International Neural Network Society (INNS) and the 2017 International Joint Conference on Neural Networks (IJCNN
- Authored by top experts, global field pioneers, and researchers working on cutting-edge applications in signal processing, speech recognition, games, adaptive control and decision-making
- Edited by high-level academics and researchers in intelligent systems and neural networks
- Includes all new chapters, including topics such as Frontiers in Recurrent Neural Network Research; Big Science, Team Science, Open Science for Neuroscience; A Model-Based Approach for Bridging Scales of Cortical Activity; A Cognitive Architecture for Object Recognition in Video; How Brain Architecture Leads to Abstract Thought; Deep Learning-Based Speech Separation and Advances in AI, Neural Networks
- Cover image
- Title page
- Table of Contents
- Copyright
- Contributors
- Editors' brief biographies
- Introduction
- 1: Advances in AI, neural networks, and brain computing: An introduction
- Abstract
- 1: Introduction
- Part 1: Fundamentals of neural networks and brain computing
- 2: Nature's learning rule: The Hebbian-LMS algorithm
- Abstract
- Acknowledgments
- 1: Introduction
- 2: Adaline and the LMS algorithm, from the 1950s
- 3: Unsupervised learning with Adaline, from the 1960s
- 4: Robert Lucky's adaptive equalization, from the 1960s
- 5: Bootstrap learning with a sigmoidal neuron
- 6: Bootstrap learning with a more “biologically correct” sigmoidal neuron
- 7: Other clustering algorithms
- 8: A general Hebbian-LMS algorithm
- 9: The synapse
- 10: Postulates of synaptic plasticity
- 11: The postulates and the Hebbian-LMS algorithm
- 12: Nature's Hebbian-LMS algorithm
- 13: Conclusion
- Appendix: Trainable neural network incorporating Hebbian-LMS learning
- Chapter postscript
- References
- Further reading
- 3: A half century of progress toward a unified neural theory of mind and brain with applications to autonomous adaptive agents and mental disorders
- Abstract
- 1: Toward a unified theory of mind and brain
- 2: A theoretical method for linking brain to mind: The method of minimal anatomies
- 3: Revolutionary brain paradigms: Complementary computing and laminar computing
- 4: The What and Where cortical streams are complementary
- 5: Adaptive resonance theory
- 6: Vector associative maps for spatial representation and action
- 7: Homologous laminar cortical circuits for all biological intelligence: Beyond Bayes
- 8: Why a unified theory is possible: Equations, modules, and architectures
- 9: All conscious states are resonant states
- 10: The varieties of brain resonances and the conscious experiences that they support
- 11: Why does resonance trigger consciousness?
- 12: Toward autonomous adaptive intelligent agents and clinical therapies in society
- References
- 4: Meaning versus information, prediction versus memory, and question versus answer
- Abstract
- Acknowledgments
- 1: Introduction
- 2: Meaning vs information
- 3: Prediction vs memory
- 4: Question vs answer
- 5: Discussion
- 6: Conclusion
- References
- 5: The brain-mind-computer trichotomy: Hermeneutic approach
- Abstract
- Acknowledgments
- 1: Dichotomies
- 2: Hermeneutics
- 3: Schizophrenia: A broken hermeneutic cycle
- 4: Toward the algorithms of neural/mental hermeneutics
- References
- Further reading
- Part 2: Brain-inspired AI systems
- 6: The new AI: Basic concepts, and urgent risks and opportunities in the internet of things
- Abstract
- 1: Introduction and overview
- 2: Brief history and foundations of the deep learning revolution
- 3: From RNNs to mouse-level computational intelligence: Next big things and beyond
- 4: Need for new directions in understanding brain and mind
- 5: Information technology (IT) for human survival: An urgent unmet challenge
- 6: From deep learning to the future: How to build and use true quantum artificial general intelligence
- Appendix: Neural network revolutions, past and future: From BP (neural and fuzzy) to quantum artificial general intelligence (QAGI)
- References
- 7: Computers versus brains: Challenges of sustainable artificial and biological intelligence
- Abstract
- Acknowledgments
- 1: The dream of creating artificial intelligence
- 2: What can AI learn from biological intelligence (BI)?
- 3: Always at the edge—The miracle of life and intelligence
- 4: Implementation of complementarity for new AI
- 5: Conclusion: Sustainable AI to support humanity
- References
- 8: Brain-inspired evolving and spiking connectionist systems
- Abstract
- Acknowledgment
- 1: The historic merge of artificial neural networks and fuzzy logic
- 2: Evolving connectionist systems (ECOS)
- 3: Spiking neural networks (SNNs)
- 4: Brain-inspired SNN. NeuCube
- 5: What role can evolutionary computation (EC) methods play for life-long learning in evolving connectionist systems (ECOS)?
- 6: Conclusion
- References
- 9: Pitfalls and opportunities in the development and evaluation of artificial intelligence systems
- Abstract
- Acknowledgment
- 1: Introduction
- 2: AI development
- 3: AI evaluation
- 4: Variability and bias in our performance estimates
- 5: Conclusion
- References
- 10: Theory of the brain and mind: Visions and history
- Abstract
- 1: Early history
- 2: Emergence of some neural network principles
- 3: Neural networks enter mainstream science
- 4: Is computational neuroscience separate from neural network theory?
- 5: Discussion
- References
- 11: From synapses to ephapsis: Embodied cognition and wearable personal assistants
- Abstract
- 1: Neural networks and neural fields
- 2: Ephapsis
- 3: Embodied cognition
- 4: Wearable personal assistants
- References
- Part 3: Cutting-edge developments in deep learning and intelligent systems
- 12: Explainable deep learning to information extraction in diagnostics and electrophysiological multivariate time series
- Abstract
- 1: Introduction
- 2: The neural network approach
- 3: Deep architectures and learning
- 4: Electrophysiological time series
- 5: Deep learning models for EEG signal processing
- 6: Future directions of research
- 7: Conclusions
- References
- 13: Computational intelligence in cyber-physical systems and the Internet of Things
- Abstract
- Acknowledgment
- 1: Introduction
- 2: System architecture
- 3: Energy harvesting and management
- 4: Learning in nonstationary environments
- 5: Model-free fault diagnosis systems
- 6: Cyber-security
- 7: Conclusions
- References
- 14: Evolving deep neural networks
- Abstract
- 1: Introduction
- 2: Background and related work
- 3: Evolution of deep learning architectures
- 4: Evolution of LSTM architectures
- 5: Application case study: Image captioning for the blind
- 6: Discussion and future work
- 7: Conclusions
- References
- 15: Evolving GAN formulations for higher-quality image synthesis
- Abstract
- 1: Introduction
- 2: Variations of GAN architectures
- 3: Evolution of loss functions
- 4: The TaylorGAN approach
- 5: Experimental setup
- 6: Results
- 7: Discussion and future work
- 8: Conclusions
- References
- 16: Multiview learning in biomedical applications
- Abstract
- 1: Introduction
- 2: Multiview learning
- 3: Multiview learning in bioinformatics
- 4: Multiview learning in neuroinformatics
- 5: Deep multimodal feature learning
- 6: Conclusions
- References
- 17: Emergence of tool construction and tool use through hierarchical reinforcement learning
- Abstract
- Acknowledgments
- 1: Introduction
- 2: Background and related works
- 3: Approach
- 4: Experiments and results
- 5: Discussion and conclusion
- References
- 18: A Lagrangian framework for learning in graph neural networks
- Abstract
- Acknowledgments
- 1: Introduction
- 2: Constraint-based models of neural networks
- 3: Local propagation in graph neural networks
- 4: Conclusions
- References
- Index
- No. of pages: 400
- Language: English
- Edition: 2
- Published: October 10, 2023
- Imprint: Academic Press
- Paperback ISBN: 9780323961042
- eBook ISBN: 9780323958165
RK
Robert Kozma
CA
Cesare Alippi
YC
Yoonsuck Choe
FM