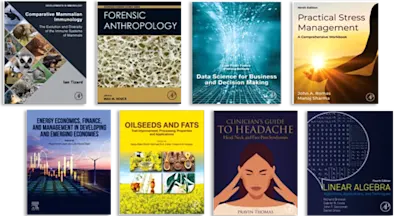
Artificial Intelligence in Pathology
Principles and Applications
- 2nd Edition - November 15, 2024
- Imprint: Elsevier
- Editors: Chhavi Chauhan, Stanley Cohen
- Language: English
- Paperback ISBN:9 7 8 - 0 - 3 2 3 - 9 5 3 5 9 - 7
- eBook ISBN:9 7 8 - 0 - 3 2 3 - 9 5 8 3 2 - 5
Artificial Intelligence in Pathology: Principles and Applications provides a strong foundation of core artificial intelligence principles and their applications in the field of… Read more
Purchase options
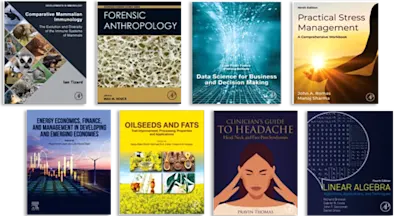
Institutional subscription on ScienceDirect
Request a sales quoteArtificial Intelligence in Pathology: Principles and Applications provides a strong foundation of core artificial intelligence principles and their applications in the field of digital pathology. This is a reference of current and emerging use of AI in digital pathology as well as the emerging utility of quantum artificial intelligence and neuromorphic computing in digital pathology. It is a must-have educational resource for lay public, researchers, academicians, practitioners, policymakers, key administrators, and vendors to stay current with the shifting landscapes within the emerging field of digital pathology. It is also of use to workers in other diagnostic imaging areas such as radiology.
This resource covers various aspects of the use of AI in pathology, including but not limited to the basic principles, advanced applications, challenges in the development, deployment, adoption, and scalability of AI-based models in pathology, the innumerous benefits of applying and integrating AI in the practice of pathology, ethical considerations for the safe adoption and deployment of AI in pathology.
- Discusses the evolution of machine learning in the field to provide a foundational background
- Addresses challenges in the development, deployment and regulation of AI in anatomic pathology
- Includes information on generative deep learning in digital pathology workflows
- Provides current tools and future perspectives
PART I PRINCIPLES
1. The evolution of machine learning
2. Basics of machine learning strategies
3. Overview of advanced neural network architectures
4. Complexity in the use of AI in anatomic pathology
5. Quantum Artificial Intelligence: Things to come
6. Dealing with data: strategies for pre-processing
7. Easing the Burden of Annotation in pathology
8. Digital path as a platform for primary diagnosis and augmentation via a deep learning
9. Challenges in the Development, Deployment, and Regulation of AI in Anatomic Pathology
10. Ethics of AI in Pathology: Current Paradigms and Emerging Issues
PART II APPLICATIONS
11. Image enhancement via AI
12. Artificial Intelligence and Cellular Segmentation in Tissue Microscopy Images
13. Precision medicine in digital pathology
14. Generative Deep Learning in Digital Pathology Workflows
15. Predictive image-based grading of human cancer
16. The interplay between tumor and immunity
17. Machine-based evaluation intra-tumoral heterogeneity and tumor-stromal interface
PART III OVERVIEW
18. The computer as digital pathology assistant
19. Neuromorphic computing, general AI, and the future of pathology
- Edition: 2
- Published: November 15, 2024
- No. of pages (Paperback): 410
- Imprint: Elsevier
- Language: English
- Paperback ISBN: 9780323953597
- eBook ISBN: 9780323958325
CC
Chhavi Chauhan
SC