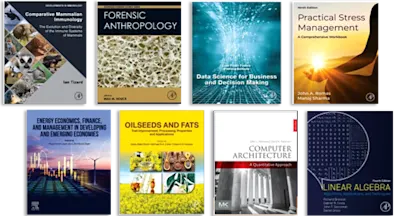
Artificial Intelligence in Manufacturing
Applications and Case Studies
- 1st Edition - January 22, 2024
- Imprint: Academic Press
- Editors: Masoud Soroush, Richard D Braatz
- Language: English
- Paperback ISBN:9 7 8 - 0 - 3 2 3 - 9 9 1 3 5 - 3
- eBook ISBN:9 7 8 - 0 - 3 2 3 - 9 9 6 7 1 - 6
Artificial Intelligence in Manufacturing: Applications and Case Studies provides detailed technical descriptions of emerging applications of AI in manufacturing using case stud… Read more
Purchase options
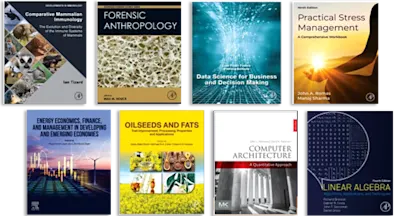
Artificial Intelligence in Manufacturing: Applications and Case Studies provides detailed technical descriptions of emerging applications of AI in manufacturing using case studies to explain implementation. Artificial intelligence is increasingly being applied to all engineering disciplines, producing insights into how we understand the world and allowing us to create products in new ways. This book unlocks the advantages of this technology for manufacturing by drawing on work by leading researchers who have successfully used it in a range of applications. Processes including additive manufacturing, pharmaceutical manufacturing, painting, chemical engineering and machinery maintenance are all addressed.
Case studies, worked examples, basic introductory material and step-by-step instructions on methods make the work accessible to a large group of interested professionals.
- Explains innovative computational tools and methods in a practical and systematic way
- Addresses a wide range of manufacturing types, including additive, chemical and pharmaceutical
- Includes case studies from industry that describe how to overcome the challenges of implementing these methods in practice
2. Machine Learning in Lithium-ion Batteries
3. Machine Learning for Emerging Two-phase Cooling Technologies
4. Algorithm-driven Design of Composite Materials Realized through Additive Manufacturing
5. Machine‐learning‐based Monitoring of Laser Powder Bed Fusion
6. Data Analytics and Cyber-physical Systems for Maintenance and Service Innovation
7. Machine Learning in Catalysis
8. Artificial Intelligence in Petrochemical Industry
9. Machine Learning-assisted Plasma Medicine
10. Dynamic Data Feature Engineering for Process Operation Troubleshooting
11. Geometric Structure-Property Relationships Captured by Theory-Guided, Interpretable Machine Learning
12. Molecular Design Blueprints from Machine Learning for Catalysts and Materials
13. Physics-driven Machine Learning for Characterizing Surface Microstructure of Complex Materials
14. Process Performance Assessment Using Machine Learning
15. Artificial Intelligence in Chemical Engineering
16. Production of Polymer Films with Optimal Properties Using Machine Learning
- Edition: 1
- Published: January 22, 2024
- Imprint: Academic Press
- Language: English
MS
Masoud Soroush
RD