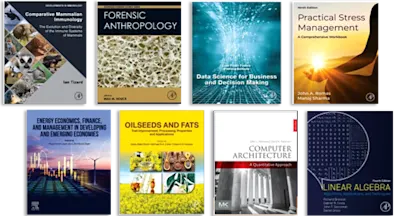
Artificial Intelligence in Biomedical and Modern Healthcare Informatics
- 1st Edition - September 27, 2024
- Imprint: Academic Press
- Editors: M. A. Ansari, R.S Anand, Pragati Tripathi, Rajat Mehrotra, Md Belal Bin Heyat
- Language: English
- Paperback ISBN:9 7 8 - 0 - 4 4 3 - 2 1 8 7 0 - 5
- eBook ISBN:9 7 8 - 0 - 4 4 3 - 2 1 8 7 1 - 2
Artificial Intelligence in Biomedical and Modern Healthcare Informatics provides a deeper understanding of the current trends in AI and machine learning within healthcare diagnosis… Read more
Purchase options
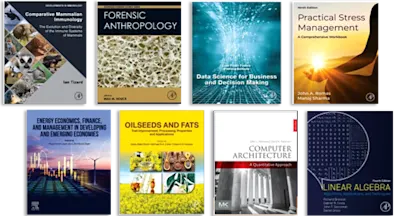
Artificial Intelligence in Biomedical and Modern Healthcare Informatics provides a deeper understanding of the current trends in AI and machine learning within healthcare diagnosis, its practical approach in healthcare, and gives insight into different wearable sensors and its device module to help doctors and their patients in enhanced healthcare system.
The primary goal of this book is to detect difficulties and their solutions to medical practitioners for the early detection and prediction of any disease.
The 56 chapters in the volume provide beginners and experts in the medical science field with general pictures and detailed descriptions of imaging and signal processing principles and clinical applications.
With forefront applications and up-to-date analytical methods, this book captures the interests of colleagues in the medical imaging research field and is a valuable resource for healthcare professionals who wish to understand the principles and applications of signal and image processing and its related technologies in healthcare.
- Discusses fundamental and advanced approaches as well as optimization techniques used in AI for healthcare systems
- Includes chapters on various established imaging methods as well as emerging methods for skin cancer, brain tumor, epileptic seizures, and kidney diseases
- Adopts a bottom-up approach and proposes recent trends in simple manner with the help of real-world examples
- Synthesizes the existing international evidence and expert opinions on implementing decommissioning in healthcare
- Promotes research in the field of health and hospital management in order to improve the efficiency of healthcare delivery systems
Graduate students and researchers on medical informatics, Healthcare workers and stakeholders involved in health technology
2. Upshots of Healthcare with AI
3. Artificial Intelligence and Machine Learning Assisted Robotic Surgery: Current Trends and Future Scope
4. A Deep Perspective of Blockchain Applications in Healthcare Sector and Industry 4.0
5. Analyzing the role of Machine Learning Techniques in Healthcare Systems
6. Artificial Intelligence (AI), Machine Learning (ML) and Deep Learning (DL) in Biomedical Fields: A Prospect in Improvising Medical Healthcare Systems
7. Artificial Intelligence in respiratory diseases with special insight through bioinformatics
8. Electroencephalography (EEG) and Epilepsy
9. A Review on Brain Computer Interface and its Applications
10. Recent Trends in Metabolomics and Artificial Intelligence
11. A comprehensive review on state of art imagined speech decoding techniques using Electroencephalography
12. Parkinson's Disease Diagnosis, Treatment, and Future Scope: An Epilogue
13. Recent Advances in Removal of Artefacts from EEG Signal Records
14. Computer Aided Diagnosis in Health Care: Case Study on Lung Cancer Diagnosis
15. AI and its role in predictive preclinical models for drug efficacy testing
16. Machine Learning-based Solutions for Brain Tumor Detection: Comparative Study and Limitations
17. Indoor and Home-Based Post-Stroke Rehabilitation Techniques- A Systemic Review
18. A comprehensive study on implementable antennas for medical applications
19. Deep Learning for Bone Age Assessment: Current Status and Future Prospects
20. Emerging Applications of Artificial Intelligence in Analyzing EEG Signals for the Healthcare Sector
21. Epilepsy Detection System using CWT and Deep-CNN
22. Isolated Indian Sign Language Recognition with Multihead Attention Transformer based network and Mediapipe’s landmarks
23. Diagnosis of Parkinson’s Disease based on Biological and Imaging-derived features using Machine learning and Deep learning
24. Brain Tumor and Feature Detection from MRI and CT scan using Artificial Intelligence
25. Neuromodulation via Brain Stimulation: A Promising Therapeutic Perspective for Alzheimer’s Disease
26. A Biosensor for the Detection of Viruses using One-Dimensional Photonic Crystals
27. Artificial Intelligence Based Seizure Detection Systems in Electroencephalography: Transforming Healthcare for Accurate Diagnosis and Treatment
28. Artificial Intelligence and Image Enhancement based methodologies used for detection of tumor in MRIs of human brain
29. Machine learning based workload Identification using Functional Near-Infrared Spectroscopy (fNIRS) Data
30. Forecasting the COVID-19 pandemic through the hybridization of Machine Intelligent Algorithms
31. Suppression of Noise Signals from Computed Tomography and Ultrasound Medical Images and Performance Evaluation
32. Prediction Of Non-Alcoholic Fat Liver Disease Using Machine Learning
33. Evaluation of Diabetes Classification with Machine Learning Framework
34. Various Segmentation Methods/ Techniques for Medical Images and The Role of IoT
35. Augmented Mass Detection of Breast Cancer in Mammogram Images Using Deep Intelligent Neural Network Model
36. CNC Machines in Production of Medical Devices
37. Analysis and prediction of Cardiomyopathy using Artificial Intelligence
38. A Preemptive Approach to Polycystic Ovary Syndrome Diagnosis using Machine Learning
39. Mapping the Landscape of Human Activity Recognition Techniques in Health Monitoring for Chronic Disease Management
40. Analysis and Organization of Mycological Skin Contaminations by Means of Medicinal Imagery
41. A Sensitive Biosensor for the Detection of Blood Components Using 2D Photonic Crystals
42. Machine Learning Assisted EEG Signal Classification for Automated Diagnosis of Mental Stress
43. CNN based Deep Learning model for Skin Cancer detection using Dermatoscopic Images
44. Bioelectrical Impedance Analysis Body Composition Estimation of Fat Mass Percentage in People with Spinal Cord Injury
45. Advanced EEG Signal Processing and Feature Extraction Concepts
46. Fractal Analysis on Biomedical Signal
47. Detection of Metastasis Osteosarcoma Using Deep Fuzzy Gradient Recurrent Convolutional Neural Network
48. Deep Learning Based Fatigue Detection Using Functional Connectivity
49. Brain Tumor Diagnosis Using Image Classifier
50. ISL Recognition System in Realtime using TensorFlow API
51. Exploring the Exciting Potential and Challenges of Brain-Computer Interfaces (BCI)
52. Transmission Dynamics of COVID-19 Virus Disease
53. Design of High Voltage Biphasic Pulse Generation Circuit with 3-Level Isolation Suitable for AED Applications
54. A Novel Scheme of Brain Tumor Detection from MRIs using K-Means Segmentation and Histogram Analysis
55. Analyzing Post COVID-19 Effects on Self-Consciousness and Awareness towards Health: A Neuroscience Framework
56. Crowdsourcing and Artificial Intelligence based Modeling Framework for effective Public Healthcare Informatics and Smart eHealth System
- Edition: 1
- Published: September 27, 2024
- Imprint: Academic Press
- Language: English
MA
M. A. Ansari
RA
R.S Anand
R. S. Anand received the B.E., M.E., and Ph.D. degrees from the University of Roorkee, Roorkee, India, in 1985, 1987, and 1992, respectively.,He is currently a Professor with the Electrical Engineering Department, IIT Roorkee, Roorkee. He has authored or coauthored more than 200 research papers in journals and conferences. His current research interests include medical signal and image processing, ultrasonic nondestructive evaluation (NDE), medical diagnosis, and speech signal processing.,Dr. Anand is a Life Member of the Ultrasonic Society of India.
PT
Pragati Tripathi
RM
Rajat Mehrotra
MH
Md Belal Bin Heyat
Md Belal Bin Heyat received the B.tech degree in E.I. from Integral University, Lucknow, UP, India in 2014. He is successfully completed Master of Technology degree in Electronics Circuit & System, department of electronics and communication engineering from Integral University, Lucknow, Uttar Pradesh, India in 2016. He has author and co-author in number of International journals, National journals, Symposium and Conferences. He is an editor and reviewer for three international and national journals. His research interests include electronics, communication engineering, instrumentation, therapy, medical and biomedical engineering.