Limited Offer
Save 50% on book bundles
Immediately download your ebook while waiting for your print delivery. No promo code needed.
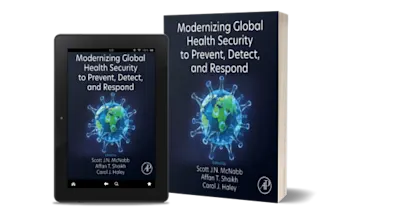
Artificial Intelligence in Bioinformatics: From Omics Analysis to Deep Learning and Network Mining reviews the main applications of the topic, from omics analysis to deep learning… Read more
Limited Offer
Artificial Intelligence in Bioinformatics: From Omics Analysis to Deep Learning and Network Mining reviews the main applications of the topic, from omics analysis to deep learning and network mining. The book includes a rigorous introduction on bioinformatics, also reviewing how methods are incorporated in tasks and processes. In addition, it presents methods and theory, including content for emergent fields such as Sentiment Analysis and Network Alignment. Other sections survey how Artificial Intelligence is exploited in bioinformatics applications, including sequence analysis, structure analysis, functional analysis, protein classification, omics analysis, biomarker discovery, integrative bioinformatics, protein interaction analysis, metabolic networks analysis, and much more.
Students and researchers in biomedicine and life science, working on bioinformatics, systems biology, molecular biology and biotechnology; computer scientists and engineers working on artificial intelligence methods and their applications in bioinformatics
MC
PG
GA
CZ
MM