Limited Offer
Save 50% on book bundles
Immediately download your ebook while waiting for your print delivery. No promo code needed.
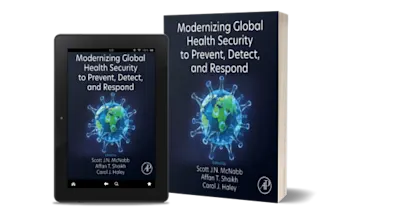
Artificial Intelligence for Neurological Disorders provides a comprehensive resource of state-of-the-art approaches for AI, big data analytics and machine learning-based neurologi… Read more
Limited Offer
Artificial Intelligence for Neurological Disorders provides a comprehensive resource of state-of-the-art approaches for AI, big data analytics and machine learning-based neurological research. The book discusses many machine learning techniques to detect neurological diseases at the cellular level, as well as other applications such as image segmentation, classification and image indexing, neural networks and image processing methods. Chapters include AI techniques for the early detection of neurological disease and deep learning applications using brain imaging methods like EEG, MEG, fMRI, fNIRS and PET for seizure prediction or neuromuscular rehabilitation.
The goal of this book is to provide readers with broad coverage of these methods to encourage an even wider adoption of AI, Machine Learning and Big Data Analytics for problem-solving and stimulating neurological research and therapy advances.
1. Early detection of neurological diseases using machine learning and deep learning techniques: A review
2. A predictive method for emotional sentiment analysis by deep learning from EEG of brainwave data
3. Machine learning and deep learning models for early-stage detection of Alzheimer's disease and its proliferation in human brain
4. Recurrent neural network model for identifying epilepsy based neurological auditory disorder
5. Recurrent neural network model for identifying neurological auditory disorder
6. Dementia diagnosis with EEG using machine learning
7. Computational methods for translational brain-behavior analysis
8. Clinical applications of deep learning in neurology and its enhancements with future directions
9. Ensemble sparse intelligent mining techniques for cognitive disease
10. Cognitive therapy for brain diseases using deep learning models
11. Cognitive therapy for brain diseases using artificial intelligence models
12. Clinical applications of deep learning in neurology and its enhancements with future predictions
13. An intelligent diagnostic approach for epileptic seizure detection and classification using machine learning
14. Neural signaling and communication using machine learning
15. Classification of neurodegenerative disorders using machine learning techniques
16. New trends in deep learning for neuroimaging analysis and disease prediction
17. Prevention and diagnosis of neurodegenerative diseases using machine learning models
18. Artificial intelligence-based early detection of neurological disease using noninvasive method based on speech analysis
19. An insight into applications of deep learning in neuroimaging
20. Incremental variance learning-based ensemble classification model for neurological disorders
21. Early detection of Parkinsons disease using adaptive machine learning techniques: A review
22. Convolutional neural network model for identifying neurological visual disorder
AA
Dr. Ajith Abraham is a Pro Vice-Chancellor at Bennette University. He is the director of Machine Intelligence Research Labs (MIR Labs), Australia. MIR Labs are a not-for-profit scientific network for innovation and research excellence connecting industry and academia. His research focuses on real world problems in the fields of machine intelligence, cyber-physical systems, Internet of things, network security, sensor networks, Web intelligence, Web services, and data mining. He is the Chair of the IEEE Systems Man and Cybernetics Society Technical Committee on Soft Computing. He is editor-in-chief of Engineering Applications of Artificial Intelligence (EAAI) and serves on the editorial board of several international journals. He received his PhD in Computer Science from Monash University, Melbourne, Australia.
SD
Sujata Dash holds the position of Professor at the Information Technology School of Engineering and Technology, Nagaland University, Dimapur Campus, Nagaland, India, bringing more than three decades of dedicated service in teaching and mentoring students. She has been honoured with the prestigious Titular Fellowship from the Association of Commonwealth Universities, United Kingdom. As a testament to her global contributions, she served as a visiting professor in the Computer Science Department at the University of Manitoba, Canada. With a prolific academic record, she has authored over 200 technical papers published in esteemed international journals, and conference proceedings, and edited book chapters by reputed publishers such as Springer, Elsevier, IEEE, IGI Global USA, and Wiley. Dr. Dash boasts ten patents, two copyrights, numerous textbooks, and edited books to her credit. Actively engaged in professional associations, she is a life member of renowned international bodies like ACM, IRSS, CSI, IMS, OITS, OMS, IACSIT, and IST, and holds a Senior membership in IEEE. Serving as a reviewer and Associate Editor for approximately 15 international journals, including prestigious publications like World Scientific, Bioinformatics, Springer, IEEE ACCESS, Inderscience, and Science Direct, she significantly contributes to the scholarly review process. Dr. Dash has been honoured with various national and international awards and serves on the editorial boards of around ten international journals. Her global presence extends to delivering keynote speeches, invited talks, and chairing special sessions at international conferences in India and overseas. Her research expertise encompasses Biomedical and Healthcare, Machine Learning, Deep Learning, Data Science, Big Data Analytics, Bioinformatics, and Intelligent Agents.
SP
Dr. Subhendu Kumar Pani received his Ph.D. from Utkal University, Odisha, India in the year 2013. He is working as a professor at Krupajal Engineering College under BPUT, Odisha, India. He has more than 20 years of teaching and research experience His research interests include Data mining, Big Data Analysis, web data analytics, Fuzzy Decision Making and Computational Intelligence. He is the recipient of 5 researcher awards. In addition to research, he has guided two PhD students and 31 M. Tech students. He has published 150 International Journal papers (100 Scopus index). His professional activities include roles as Book Series Editor (CRC Press, Apple Academic Press, Wiley-Scrivener), Associate Editor, Editorial board member and/or reviewer of various International Journals. He is an Associate with no. of the conference societies. He has more than 250 international publications, 5 authored books, 25 edited and upcoming books; 40 book chapters into his account. He is a fellow in SSARSC and a life member in IE, ISTE, ISCA, and OBA.OMS, SMIACSIT, SMUACEE, CSI.
LG