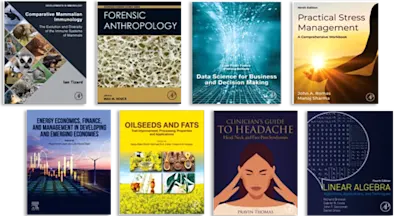
Artificial Intelligence and Data Driven Optimization of Internal Combustion Engines
- 1st Edition - January 5, 2022
- Imprint: Elsevier
- Editors: Jihad Badra, Pinaki Pal, Yuanjiang Pei, Sibendu Som
- Language: English
- Paperback ISBN:9 7 8 - 0 - 3 2 3 - 8 8 4 5 7 - 0
- eBook ISBN:9 7 8 - 0 - 3 2 3 - 8 8 4 5 8 - 7
Artificial Intelligence and Data Driven Optimization of Internal Combustion Engines summarizes recent developments in Artificial Intelligence (AI)/Machine Learning (ML) and data d… Read more
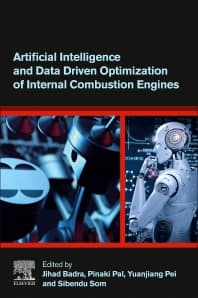
Purchase options
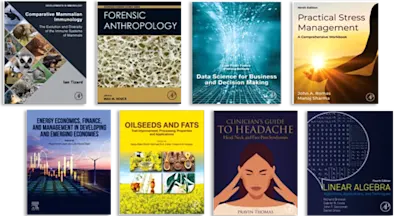
Institutional subscription on ScienceDirect
Request a sales quoteArtificial Intelligence and Data Driven Optimization of Internal Combustion Engines summarizes recent developments in Artificial Intelligence (AI)/Machine Learning (ML) and data driven optimization and calibration techniques for internal combustion engines. The book covers AI/ML and data driven methods to optimize fuel formulations and engine combustion systems, predict cycle to cycle variations, and optimize after-treatment systems and experimental engine calibration. It contains all the details of the latest optimization techniques along with their application to ICE, making it ideal for automotive engineers, mechanical engineers, OEMs and R&D centers involved in engine design.
- Provides AI/ML and data driven optimization techniques in combination with Computational Fluid Dynamics (CFD) to optimize engine combustion systems
- Features a comprehensive overview of how AI/ML techniques are used in conjunction with simulations and experiments
- Discusses data driven optimization techniques for fuel formulations and vehicle control calibration
Automotive and Mechanical Engineers in industry and academia. OEMs and those in IC Engine R&D
- Cover image
- Title page
- Table of Contents
- Copyright
- Contributors
- Foreword
- Preface
- Chapter 1. Introduction
- 1. Industrial revolution
- 2. Artificial intelligence, machine learning, and deep learning
- 3. Machine learning algorithms
- 4. Artificial intelligence–based fuel-engine co-optimization
- 5. Summary
- Section 1. Artificial Intelligence to optimize fuel formulation
- Chapter 2. Optimization of fuel formulation using adaptive learning and artificial intelligence
- 1. Introduction and motivation
- 2. Mixed-mode combustion and fuel performance metrics
- 3. A neural network model to predict fuel research octane numbers
- 4. Optimization problem formulation and description of solution approaches
- 5. Numerical experiments and results
- 6. Discussion
- 7. Summary and concluding remarks
- Chapter 3. Artificial intelligence–enabled fuel design
- 1. Transportation fuels
- 2. Application of artificial intelligence to fuel formulation
- 3. Conclusions and perspectives
- Section 2. Artificial Intelligence and computational fluid dynamics to optimize internal combustion engines
- Chapter 4. Engine optimization using computational fluid dynamics and genetic algorithms
- 1. Introduction
- 2. Modeling framework and acceleration strategies
- 3. Optimization methods
- 4. Summary and concluding remarks
- Chapter 5. Computational fluid dynamics–guided engine combustion system design optimization using design of experiments
- 1. Introduction
- 2. Methodologies
- 3. A recent application
- 4. Recommendations for best practice
- 5. Conclusions and perspectives
- Chapter 6. A machine learning-genetic algorithm approach for rapid optimization of internal combustion engines
- 1. Introduction
- 2. Engine optimization problem setup
- 3. Training and data examination
- 4. Machine learning-genetic algorithm approach
- 5. Automated machine learning-genetic algorithm
- 6. Summary
- Chapter 7. Machine learning–driven sequential optimization using dynamic exploration and exploitation
- 1. Introduction
- 2. Active ML optimization (ActivO)
- 3. Case study 1: two-dimensional cosine mixture function
- 4. Case study 2: computational fluid dynamics (CFD)-based engine optimization
- 5. Conclusions
- Section 3. Artificial Intelligence to predict abnormal engine phenomena
- Chapter 8. Artificial-intelligence-based prediction and control of combustion instabilities in spark-ignition engines
- 1. Introduction
- 2. Case study: artificial-intelligence-enhanced modeling of dilute spark-ignition cycle-to-cycle variability
- 3. Case study: neural networks for combustion stability control
- 4. Case study: learning reference governor for model-free dilute limit identification and avoidance
- 5. Summary
- Chapter 9. Using deep learning to diagnose preignition in turbocharged spark-ignited engines
- 1. Introduction
- 2. Preignition detection using machine learning algorithm
- 3. Activation functions
- 4. Experiments and data extraction
- 5. Machine learning methodology
- 6. Model 1: Input from principal component analysis
- 7. Model 2: Time series input
- 8. Model metrics
- 9. Results and discussion
- 10. Conclusions
- Index
- Edition: 1
- Published: January 5, 2022
- No. of pages (Paperback): 260
- No. of pages (eBook): 260
- Imprint: Elsevier
- Language: English
- Paperback ISBN: 9780323884570
- eBook ISBN: 9780323884587
JB
Jihad Badra
Jihad Badra is the Engine Combustion Team leader in the Transport Technologies Research and Development Division at Saudi Aramco. He joined Saudi Aramco in 2014 after working as a Postdoctoral Researcher in the Clean Combustion Research Center at King Abdullah University of Science and Technology (KAUST). Jihad’s research interest is in developing and optimizing internal combustion engine technologies with decreased net environmental impact. Jihad’s current focus is on fuel formulation for advanced engines and engine modelling using computational fluid dynamics. Jihad has more than 50 peer-reviewed journal papers. Jihad received his BASc in Mechanical Engineering from the University of Balamand, Lebanon and MASc and PhD degrees in Combustion Research in Mechanical Engineering at the University of Sydney, Australia.
Affiliations and expertise
Team Leader, Transport Technologies Research and Development Division, Saudi Aramco, Saudi ArabiaPP
Pinaki Pal
Pinaki Pal is a research scientist in Argonne’s Energy Systems division. His research interests broadly lie in the areas of computational fluid dynamics (CFD), turbulent combustion modeling, machine learning, computational science, and high-performance computing, for a wide range of applications, such as propulsion (automotive and aerospace) and material synthesis. Dr. Pal received his PhD from University of Michigan-Ann Arbor (2015) in Mechanical Engineering, with specialization in turbulent combustion modeling and CFD for low temperature combustion applications in both internal combustion engines and gas turbines. He also holds a Bachelor of Technology in Mechanical Engineering from the Indian Institute of Technology Kharagpur (India) (2011).
Affiliations and expertise
Research Scientist, Argonne National Laboratory, IL, USAYP
Yuanjiang Pei
Yuanjiang Pei is a Technical Specialist at Aramco Americas: Aramco Research Center – Detroit working on the co-optimization of fuels and engines in pursuit of higher internal combustion engine efficiency. Pei has more than 10 years of experience working in the engine research and automotive industry. He joined Aramco in late 2015 after previously working more than 2 years on engine combustion modeling at Argonne National Laboratory and 5 years on engine management system calibration and project management at Delphi. Pei is actively involved in the organization of several international conferences, including Society of Automotive Engineers (SAE) World Congress, American Society of Mechanical Engineers (ASME) Internal Combustion Engine Fall (ICEF) Conference and Engine Combustion Network (ECN) workshops.
Affiliations and expertise
Technical Specialist, Aramco Americas: Aramco Research Center - Detroit, Michigan, USASS
Sibendu Som
Sibendu Som is the manager of the Computational Multi-Physics Research Section in the Energy Systems Division at Argonne National Laboratory and a senior scientist at the Consortium for Advanced Science and Engineering, University of Chicago. Dr. Som has over a decade of experience in enabling technologies for more efficient engine combustion using computational tools. He leads a Computational Fluid Dynamics (CFD) team at Argonne National Laboratory with a research focus on the development of nozzle-flow, spray, and combustion models, using high-performance computing (HPC) for internal combustion engine (ICE) applications. His team is responsible for developing predictive simulation capabilities to enable OEMs to develop advanced high-efficiency, low-emission engines. Dr. Som’s group is pioneering the implementation of machine learning (ML) techniques to further speed up piston engine and gas turbine simulations. He is a co-founder and technical lead of Argonne’s Virtual Engine Research Institute and Fuels Initiative (VERIFI) program, which is aimed at providing predictive simulations for industry. Dr. Som and his team are recognized worldwide for improving the predictive capability of simulation tools and applying these tools using HPC to reduce time to design.
Affiliations and expertise
Manager, Computational Multi-Physics Research Section, Energy Systems Division, Argonne National Laboratory, Lemont, USARead Artificial Intelligence and Data Driven Optimization of Internal Combustion Engines on ScienceDirect