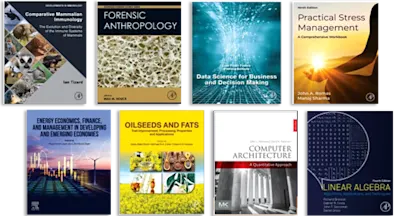
Applied Statistical Methods
- 1st Edition - May 10, 2014
- Imprint: Academic Press
- Author: Irving W. Burr
- Editor: J. William Schmidt
- Language: English
- Paperback ISBN:9 7 8 - 1 - 4 8 3 2 - 3 6 8 0 - 3
- eBook ISBN:9 7 8 - 1 - 4 8 3 2 - 7 7 8 6 - 8
Applied Statistical Methods covers the fundamental understanding of statistical methods necessary to deal with a wide variety of practical problems. This 14-chapter text presents… Read more
Purchase options
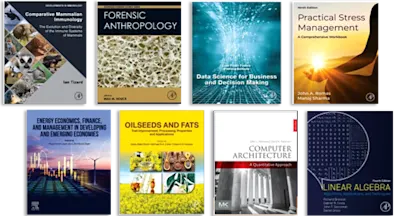
Applied Statistical Methods covers the fundamental understanding of statistical methods necessary to deal with a wide variety of practical problems. This 14-chapter text presents the topics covered in a manner that stresses clarity of understanding, interpretation, and method of application. The introductory chapter illustrates the importance of statistical analysis. The next chapters introduce the methods of data summarization, including frequency distributions, cumulative frequency distributions, and measures of central tendency and variability. These topics are followed by discussions of the fundamental principles of probability, the concepts of sample spaces, outcomes, events, probability, independence of events, and the characterization of discrete and continuous random variables. Other chapters explore the distribution of several important statistics; statistical tests of hypotheses; point and interval estimation; and simple linear regression. The concluding chapters review the elements of single- and two-factor analysis of variance and the design of analysis of variance experiments. This book is intended primarily for advanced undergraduate and graduate students in the mathematical, physical, and engineering sciences, as well as in economics, business, and related areas. Researchers and line personnel in industry and government will find this book useful in self-study.
Preface
Acknowledgments
Chapter 1 Introduction
1.1 Why Statistical Methods?
1.2 Advice to the Student
Chapter 2 The Frequency Distribution—A Tool and a Concept
2.1 Introduction
2.2 An Example of a Frequency Distribution
2.3 Frequency Class Nomenclature and Tabulation
2.4 Discrete versus Continuous Data
2.5 Graphical Representation of a Frequency Distribution
2.6 The Cumulative Frequency Graph
2.7 What a Frequency Distribution Shows
2.8 Some Examples of Use of Frequency Tables and Graphs
2.9 Sample versus Population
2.10 Summary
Problems
Chapter 3 Summarization of Data by Objective Measures
3.1 Introduction
3.2 Some Averages
3.3 Some Measures of Variability
3.4 Efficient Calculation of Averages and Standard Deviations
3.4.1 Calculations for Frequency Data
3.5 Further Descriptive Measures of Frequency Distributions, Third and Fourth Moments
3.6 Summary
Problems
Chapter 4 Some Elementary Probability
4.1 Introduction
4.2 Sample Spaces of Outcomes
4.3 Events
4.3.1 Relations of Events
4.3.2 Combinations of Events
4.4 Probabilities of Events
4.5 Probabilities on Discrete Sample Spaces
4.5.1 Countably Infinite Spaces
4.5.2 Events over Discrete Spaces
4.6 Independent and Dependent Events
4.6.1 Conditional Probabilities
4.6.2 Repeated Trials
4.7 Discrete Probabilities
4.7.1 Permutations and Combinations
4.7.2 Discrete Probability Examples
4.8 Probabilities on Continuous Spaces
4.9 Applied Bayes' Probabilities—Posterior Probabilities
4.10 Interpretation of a Probability
4.11 Random Variables
4.12 Summary
Problems
Chapter 5 Some Discrete Probability Distributions
5.1 Theoretical Populations
5.2 Discrete Probability Distributions in General
5.2.1 Expected Values for Y and Functions of Y
5.2.2 Population Curve-Shape Characteristics
5.2.3 Algebra of Expectations
5.2.4 Further on Population Moments
5.3 The Binomial Distribution
5.3.1 Examples of the Binomial Distribution
5.3.2 Population Moments for Binomial Distributions
5.3.3 Use of Binomial Tables
5.3.4 Calculation of a Binomial Distribution
5.3.5 Approximations to the Binomial Distribution
5.3.6 Conditions of Applicability of the Binomial Distribution
5.4 The Poisson Distribution
5.4.1 A Derivation of the Poisson Probability Function
5.4.2 Examples of the Poisson Distribution
5.4.3 Tables of the Poisson Distribution
5.4.4 Using the Poisson Distribution to Approximate the Binomial
5.4.5 Derivation of the Poisson as a Limit of the Binomial
5.4.6 Conditions of Applicability of the Poisson Distribution
5.5 The Hypergeometric Distribution
5.5.1 Tables for the Hypergeometric Distribution
5.5.2 Examples of the Hypergeometric Distribution
5.5.3 Moments for the Hypergeometric Distribution
5.5.4 Binomial Approximations to the Hypergeometric Distribution
5.5.5 Poisson Approximations to the Hypergeometric Distribution
5.5.6 Approximations to Sums of Terms of the Hypergeometric Distribution
5.5.7 Conditions of Applicability of the Hypergeometric Distribution
5.5.8 Applications of the Hypergeometric Distribution
5.6 The Uniform Distribution
5.7 The Geometric Distribution
5.8 The Negative Binomial Distribution
5.9 Generating Samples from Discrete Distributions
5.10 Summary
References
Problems
Chapter 6 Some Continuous Probability Distributions
6.1 Continuous Probability Distributions
6.2 Some General Properties of Continuous Distributions
6.2.1 Moments for a Continuous Distribution
6.3 The Normal Curve
6.3.1 Properties of the Normal Distribution
6.3.2 The General Normal Curve
6.3.3 Sketching a Normal Curve
6.3.4 Approximating Probabilities by a Normal Distribution
6.4 The Rectangular Distribution
6.5 The Exponential Distribution
6.6 The Gamma Distribution
6.6.1 Tables of the Gamma Distribution
6.6.2 Relation to the Normal Distribution
6.6.3 Use of the Gamma Distribution to Approximate Discrete Distributions
6.7 The Beta Distribution
6.8 The Weibull Distribution
6.9 The Pearson System of Distributions
6.10 An Easily Fitted General System of Frequency Curves
6.11 Sums and Averages and a Central Limit Theorem
6.12 Tchebycheff's Theorem
6.13 Summary
6.14 Proofs of Some Relations in Section 6.11
References
Problems
Chapter 7 Some Sampling Distributions
7.1 Distribution of Sample Statistics from Populations
7.2 Choice of Sample
7.2.1 Sampling from a Probability Distribution
7.2.2 Machine Generation of Random Samples
7.3 Sampling Distributions of a Sample Statistic
7.4 Distribution of Sample Means
7.4.1 Standardized Distribution for Means
7.4.2 Distribution of Means when Standard Deviation Is Unknown
7.4.3 Areas for the t Distribution
7.4.4 Interpolation Note
7.4.5 Distribution of Means from Nonnormal Populations
7.5 Distribution of Sample Variances
7.5.1 Distribution of Sample Standard Deviation
7.5.2 Population of y's Nonnormal
7.5.3 Tables of Chi-Square
7.6 Joint Distribution of ȳ and s from a Normal Population
7.7 Two Normal Populations, Independent Samples
7.7.1 Sum and Difference of Two Means, Standard Deviations Known
7.7.2 Sums and Differences of Two Means, Standard Deviations Unknown but Equal
7.7.3 Two Variances, F Distribution
7.7.4 Two Variances, Large Samples
7.8 Sampling Aspects of the Binomial and Poisson Distributions
7.9 Sum of Two Independent Chi-Square Variables
7.10 Noncentral Distributions
7.11 Summary
References
Problems
Chapter 8 Statistical Tests of Hypotheses—General and One Sample
8.1 Introduction
8.2 An Example
8.2.1 Approach 1 Given n, Set Significance Level α
8.2.2 Approach 2 Set Two Risks: α and α βμ, and Find n
8.3 Summary of the Elements in Tests of Hypotheses on One Parameter
8.4 Summary of Significance Testing for One Mean with σ Unknown
8.5 Interpretation of Decisions in Hypothesis Testing
8.6 Nonnormal Populations of y's
8.7 Significance Testing for Mean μ, with σ Unknown
8.7.1 Example
8.7.2 Operating Characteristic Curve
8.8 Significance Tests for Variability
8.8.1 An Example of First Approach
8.8.2 The Second Approach of 4, in Section 8.8
8.8.3 Operating Characteristic Curves for Variability Tests
8.8.4 Large Samples
8.9 Significance Testing for Attributes
8.9.1 The Binomial Tests
8.9.2 The Poisson Tests
8.9.3 Other Attribute Distributions
8.9.4 Operating Characteristic Curves
8.10 Relation of Significance Testing to Decision Theory
8.11 Summary
References
Problems
Chapter 9 Significance Tests—Two Samples
9.1 The General Problem
9.2 Tests on Two Variances—The F Test
9.2.1 An Example
9.2.2 Large Sample Tests
9.2.3 A Large Sample Example
9.3 Differences between Means
9.3.1 Standard Deviations Known
9.3.2 Standard Deviations Equal but Unknown
9.3.3 Standard Deviations Unknown and Possibly Unequal
9.4 Significance of Differences—Binomial Data
9.5 Significance of Differences—Poisson Data
9.5.1 Unequal Areas of Opportunity
9.6 Matched Pair Data. Importance of Experimental Design
9.6.1 Matched-Pair Model
9.7 Sample Sizes Needed for Tests of Two Means
9.8 Summary
References
Problems
Chapter 10 Estimation of Population Characteristics
10.1 Point Estimates—General Idea
10.2 Which Estimator to Use—Characteristics of Estimation
10.2.1 Consistency and Sufficiency
10.3 How to Find a Desirable Estimator
10.4 Point Estimates—Common Cases
10.5 Interval Estimation in General
10.5.1 Geometrical Argument for Confidence Intervals
10.6 Confidence Intervals for μ
10.7 Confidence Intervals for σ
10.7.1 Large Confidence Limits for σ
10.8 How to Have Narrower Confidence Intervals
10.9 Confidence Intervals for Functions of Two Parameters—Two Samples
10.9.1 Confidence Limits on the Difference of Means
10.9.2 Confidence Limits on the Ratio of σ's
10.9.3 Paired Differences
10.10 Confidence Limits for Attribute Data
10.10.1 Exact Method for Binomial Population
10.10.2 Normal Approximation for Confidence Limits for the Binomial
10.10.3 Exact Method for Poisson Population
10.10.4 Normal Approximation for Confidence Limits for the Poisson
10.10.5 Tables of Confidence Limits
10.10.6 Confidence Limits for Two Samples of Attribute Data
10.11 Relation between Interval Estimation and Significance Testing
10.12 Summary
References
Problems
Chapter 11 Simple Regression
11.1 Regression, A Study of Relationship
11.2 The Scatter Diagram
11.3 Line of Best Fit to "Linear" Data
11.3.1 Least Squares Fitting
11.3.2 Calculational Aspects
11.3.3 The Linear Model and Its Parameters
11.4 Sampling Distributions for Estimates
11.5 Significance Tests and Confidence Intervals for Parameters in Linear Regression
11.5.1 Slope
11.5.2 Intercept
11.5.3 Mean of Y's: μY
11.5.4 Error Variance σε2, and σε
11.5.5 Regression Line Mean: μY.x = μY + β(X - X̄)
11.6 Correlational Aspects
11.7 Grouped Bivariate Data
11.8 Special Case μY.x = β1X
11.9 Significance of Differences between Two Slopes
11.10 Nonlinearity Test
11.11 Use of Least Squares Fitting for Other Trends
11.11.1 Functions Linear in the Parameters
11.11.2 Least Squares after a Transformation
11.11.3 Intrinsically Nonlinear Cases
11.12 Applications to Industry and the Laboratory
11.13 Summary
References
Problems
Chapter 12 Simple Analysis of Variance
12.1 General Concept of Analysis of Variance
12.2 One-Factor Analysis of Variance
12.2.1 The Model
12.2.2 The Formulas and Test
12.2.3 The Case of Unequal Sample Sizes
12.2.4 Orthogonal Contrasts
12.3 Orthogonal Polynomials and Tests
12.4 A Method of Multiple Contrasts
12.4.1 The Newman-Keuls Multiple Range Test
12.4.2 Example
12.4.3 Interpretation of Risk α
12.5 Testing Homogeneity of Variances
12.5.1 An Example
12.5.2 Q Test with Unequal Degrees of Freedom
12.5.3 Q Test for Ranges
12.6 Types of Factors
12.7 Analysis of Variance for Two Factors
12.7.1 An Example
12.7.2 The Models and Assumptions
12.7.3 Expected Mean Squares and Significance Tests
12.7.4 Interpretation of Significant Factors
12.7.5 Case of Unreplicated Two-Factor Experiments
12.7.6 Example of an Unreplicated Two-Factor Completely Randomized Design
12.8 Other Models
12.9 Summary
References
Problems
Chapter 13 Multiple Regression
13.1 Introduction
13.2 First Approach
13.2.1 Data Table Format
13.2.2 Fitting the Equation
13.2.3 Alternative Forms of Normal Equations and Regression
13.2.4 Describing Goodness of Fit
13.2.5 Systematic Solution of the Normal Equations
13.2.6 Significance Tests on the Explained Variation
13.2.7 Simple Example
13.2.8 Second Example
13.3 Second Approach
13.3.1 Vectors and Matrices
13.3.2 The Matrix Approach
13.3.3 Selection of a Set of Predictors
13.3.4 Calculation of an Inverse
13.4 Summary of Approach
13.5 Adequacy of Regression Model
13.6 Comments and Precautions
References
Problems
Chapter 14 Goodness of Fit Tests, Contingency Tables
14.1 Introduction
14.2 The Chi-square Test for Cell Frequencies, Observed versus Theoretical
14.2.1 An Example
14.3 Testing Goodness of Fit of Theoretical Distributions
14.3.1 A Binomial Example
14.3.2 Examples of Tests of Normality
14.3.3 Example of a Gamma Distribution Fit
14.3.4 Example of a Poisson Distribution
14.4 Other Goodness of Fit Tests
14.5 Contingency Tables
14.5.1 An Example
14.5.2 The General Setup of a Contingency Table
14.5.3 A 2 ∙ 2 Contingency Table
14.5.4 Case of a 2 • b Contingency Table
14.6 The Sign Test
14.7 Summary
References
Problems
Appendix
Answers to Odd-Numbered Problems
Index
- Edition: 1
- Published: May 10, 2014
- Imprint: Academic Press
- Language: English
Read Applied Statistical Methods on ScienceDirect