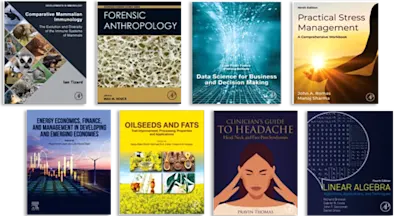
Applications of Deep Machine Learning in Future Energy Systems
- 1st Edition - August 20, 2024
- Imprint: Elsevier
- Editor: Mohammad-Hassan Khooban
- Language: English
- Paperback ISBN:9 7 8 - 0 - 4 4 3 - 2 1 4 3 2 - 5
- eBook ISBN:9 7 8 - 0 - 4 4 3 - 2 1 4 3 1 - 8
Applications of Deep Machine Learning in Future Energy Systems pushes the limits of current Artificial Intelligence techniques to present deep machine learning suitable for the c… Read more
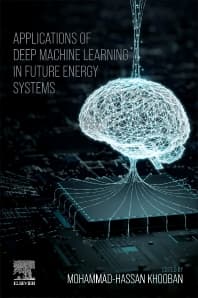
Purchase options
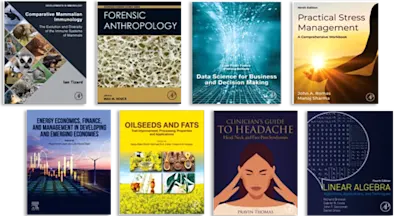
Institutional subscription on ScienceDirect
Request a sales quoteApplications of Deep Machine Learning in Future Energy Systems pushes the limits of current Artificial Intelligence techniques to present deep machine learning suitable for the complexity of sustainable energy systems. The first two chapters take the reader through the latest trends in power engineering and system design and operation before laying out current AI approaches and limitations. Later chapters provide in-depth accounts of specific challenges and the use of innovative third-generation machine learning, including neuromorphic computing, to resolve issues from security to power supply.
An essential tool for the management, control, and modelling of future energy systems, this book maps a practical path towards AI capable of supporting sustainable energy.
- Clarifies the current state and future trends of energy system machine learning and the pitfalls facing our transitioning systems
- Provides guidance on 3rd-generation AI tools for meeting the challenges of modeling and control in modern energy systems
- Includes case studies and practical examples of potential applications to inspire and inform researchers and industry developers
2. Artificial intelligence and Machine learning in Future Energy Systems (State-of-Art, future development)
Jalal Heidary
3. Digital Twins-Assisted Design of Next-Generation DC Microgrid
Meysam Gheisarnejad, Maryam Homayounzadeh, Burak Yildirim
4. Deep Learning-Based Procedure for Profit Maximization of EV Charging Stations
Mohammad Hassan Khooban, Peyman Razmi, MASOUMEH SEYEDYAZDI
5. Deep Frequency Control of Power Grids Under Cyber Attacks
Mohammad Aghamohammadi, jalal heidary, Soroush Oshnoei
6. Application of Q-Learning in Stabilization of Multi Carrier Energy Systems
Meysam Gheisarnejad, Maryam Homayounzadeh, Burak Yildirim
7. Design of Next-Generation of 5G Data Center Power Supply based on AI
Mohammad Hassan Khooban, Meysam Gheisarnejad
8. Smart EV Battery Charger Based on Deep Machine Learning
Mohammad Hassan Khooban, Jalil Boudjadar, Mehdi Rafiei
9. Machine learning in Talkative Power
Mohammad Hassan Khooban, Zahra Ghahraman Izadi, Ali Mousavi
10. Advanced Control of Power Electronics-based Machine Learning
Maryam Homayounzadeh, Meysam Gheisarnejad, Mohamadreza Homayounzade, Mohammad Hassan Khooban
11. Multi-Level Energy Management and Optimal Control System in Smart Cities Based on Deep Machine Learning
Javid Ghafourian, Atefe Hedayatnia, Ahmed Al-Durra, Reza Sepehrzad
- Edition: 1
- Published: August 20, 2024
- No. of pages (Paperback): 334
- Imprint: Elsevier
- Language: English
- Paperback ISBN: 9780443214325
- eBook ISBN: 9780443214318
MK