Limited Offer
Save 50% on book bundles
Immediately download your ebook while waiting for your print delivery. No promo code needed.
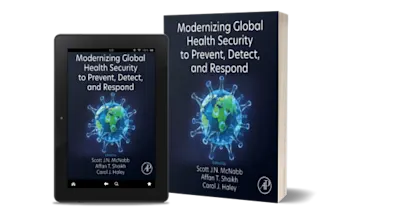
Applications of Artificial Intelligence in Medical Imaging provides the description of various biomedical image analysis in disease detection using AI that can be used to incorpora… Read more
Limited Offer
Applications of Artificial Intelligence in Medical Imaging provides the description of various biomedical image analysis in disease detection using AI that can be used to incorporate knowledge obtained from different medical imaging devices such as CT, X-ray, PET and ultrasound. The book discusses the use of AI for detection of several cancer types, including brain tumor, breast, pancreatic, rectal, lung colon, and skin. In addition, it explains how AI and deep learning techniques can be used to diagnose Alzheimer's, Parkinson's, COVID-19 and mental conditions.
This is a valuable resource for clinicians, researchers and healthcare professionals who are interested in learning more about AI and its impact in medical/biomedical image analysis.
1. Introduction to artificial intelligence techniques for medical image analysis
ABDULHAMIT SUBASI
2. Lung cancer detection from histopathological lung tissue images using deep learning
AAYUSH RAJPUT AND ABDULHAMIT SUBASI
3. Magnetic resonance imagining-based automated brain tumor detection using deep learning techniques
ABHRANTA PANIGRAHI AND ABDULHAMIT SUBASI
4. Breast cancer detection from mammograms using artificial intelligence
ABDULHAMIT SUBASI, AAYUSH DINESH KANDPAL, KOLLA ANANT RAJ AND ULAS BAGCI
5. Breast tumor detection in ultrasound images using artificial intelligence
OMKAR MODI AND ABDULHAMIT SUBASI
6. Artificial intelligence-based skin cancer diagnosis
ABDULHAMIT SUBASI AND SAQIB AHMED QURESHI
7. Brain stroke detection from computed tomography images using deep learning algorithms
AYKUT DIKER, ABDULLAH ELEN AND ABDULHAMIT SUBASI
8. A deep learning approach for COVID-19 detection from computed tomography scans
ASHUTOSH VARSHNEY AND ABDULHAMIT SUBASI
9. Detection and classification of Diabetic Retinopathy Lesions using deep learning
SIDDHESH SHELKE AND ABDULHAMIT SUBASI
10. Automated detection of colon cancer using deep learning
AAYUSH RAJPUT AND ABDULHAMIT SUBASI
11. Brain hemorrhage detection using computed tomography images and deep learning
ABDULLAH ELEN, AYKUT DIKER AND ABDULHAMIT SUBASI
12. Artificial intelligence-based retinal disease classification using optical coherence tomography images
SOHAN PATNAIK AND ABDULHAMIT SUBASI
13. Diagnosis of breast cancer from histopathological images with deep learning architectures
EMRAH HANCER AND ABDULHAMIT SUBASI
14. Artificial intelligence based Alzheimer’s disease detection using deep feature extraction
MANAV NITIN KAPADNIS, ABHIJIT BHATTACHARYYA AND ABDULHAMIT SUBASI
AS
Abdulhamit Subasi is a highly specialized expert in the fields of Artificial Intelligence, Machine Learning, and Biomedical Signal and Image Processing. His extensive expertise in applying machine learning across diverse domains is evident in his numerous contributions, including the authorship of multiple book chapters, as well as the publication of a substantial body of research in esteemed journals and conferences. His career has spanned various prestigious institutions, including the Georgia Institute of Technology in Georgia, USA, where he served as a dedicated researcher. In recognition of his outstanding research contributions, Subasi received the prestigious Queen Effat Award for Excellence in Research in May 2018. His academic journey includes a tenure as a Professor of computer science at Effat University in Jeddah, Saudi Arabia, from 2015 to 2020. Since 2020, he has assumed the role of Professor of medical physics at the Faculty of Medicine, University of Turku in Turku, Finland