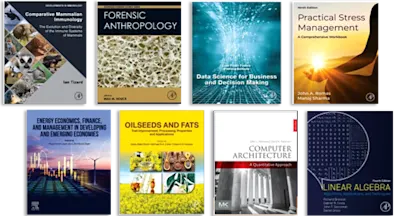
AI Assurance
Towards Trustworthy, Explainable, Safe, and Ethical AI
- 1st Edition - October 17, 2022
- Imprint: Academic Press
- Editors: Feras A. Batarseh, Laura Freeman
- Language: English
- Paperback ISBN:9 7 8 - 0 - 3 2 3 - 9 1 9 1 9 - 7
- eBook ISBN:9 7 8 - 0 - 3 2 3 - 9 1 8 8 2 - 4
AI Assurance: Towards Trustworthy, Explainable, Safe, and Ethical AI provides readers with solutions and a foundational understanding of the methods that can be applied to test A… Read more
Purchase options
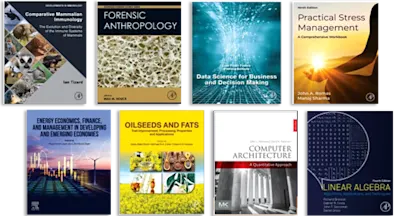
AI Assurance: Towards Trustworthy, Explainable, Safe, and Ethical AI provides readers with solutions and a foundational understanding of the methods that can be applied to test AI systems and provide assurance. Anyone developing software systems with intelligence, building learning algorithms, or deploying AI to a domain-specific problem (such as allocating cyber breaches, analyzing causation at a smart farm, reducing readmissions at a hospital, ensuring soldiers’ safety in the battlefield, or predicting exports of one country to another) will benefit from the methods presented in this book.
As AI assurance is now a major piece in AI and engineering research, this book will serve as a guide for researchers, scientists and students in their studies and experimentation. Moreover, as AI is being increasingly discussed and utilized at government and policymaking venues, the assurance of AI systems—as presented in this book—is at the nexus of such debates.
- Provides readers with an in-depth understanding of how to develop and apply Artificial Intelligence in a valid, explainable, fair and ethical manner
- Includes various AI methods, including Deep Learning, Machine Learning, Reinforcement Learning, Computer Vision, Agent-Based Systems, Natural Language Processing, Text Mining, Predictive Analytics, Prescriptive Analytics, Knowledge-Based Systems, and Evolutionary Algorithms
- Presents techniques for efficient and secure development of intelligent systems in a variety of domains, such as healthcare, cybersecurity, government, energy, education, and more
- Covers complete example datasets that are associated with the methods and algorithms developed in the book
2. Setting the goals for ethical, unbiased and fair AI
3. An overview of explainable and interpretable AI
4. Bias, Fairness, and assurance in AI: Overview and Synthesis
5. An evaluation of the potential global impacts of AI assurance
6. The role of inference in AI: start S.M.A.L.L. with mindful models
7. Outlier detection using AI: a survey
8. AI assurance using casual inference: application to public policy
9. Data collection, wrangling and preprocessing for AI assurance
10. Coordination-aware assurance for end-to-end machine learning systems: the R3E approach
11. Assuring AI methods for economic policymaking
12. Panopticon implications of ethical AI: equity, disparity, and inequality in healthcare
13. Recent advances in uncertainty quantification methods for engineering problems
14. Socially responsible AI assurance in precision agriculture for farmers and policymakers
15. The application of AI assurance in precision farming and agricultural economics
16. Bringing dark data to light with AI for evidence-based policy making
- Edition: 1
- Published: October 17, 2022
- Imprint: Academic Press
- Language: English
FB
Feras A. Batarseh
LF