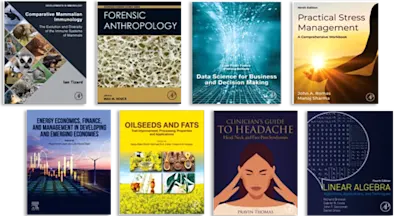
Advances in Subsurface Data Analytics
- 1st Edition - May 18, 2022
- Imprint: Elsevier
- Editors: Shuvajit Bhattacharya, Haibin Di
- Language: English
- Paperback ISBN:9 7 8 - 0 - 1 2 - 8 2 2 2 9 5 - 9
- eBook ISBN:9 7 8 - 0 - 1 2 - 8 2 2 3 0 8 - 6
Advances in Subsurface Data Analytics: Traditional and Physics-Based Approaches brings together the fundamentals of popular and emerging machine learning (ML) algorithms with thei… Read more
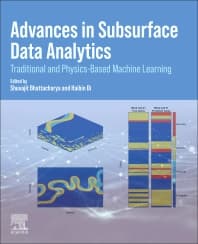
Purchase options
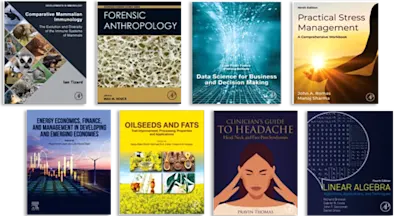
Institutional subscription on ScienceDirect
Request a sales quoteAdvances in Subsurface Data Analytics: Traditional and Physics-Based Approaches brings together the fundamentals of popular and emerging machine learning (ML) algorithms with their applications in subsurface analysis, including geology, geophysics, petrophysics, and reservoir engineering. The book is divided into four parts: traditional ML, deep learning, physics-based ML, and new directions, with an increasing level of diversity and complexity of topics. Each chapter focuses on one ML algorithm with a detailed workflow for a specific application in geosciences. Some chapters also compare the results from an algorithm with others to better equip the readers with different strategies to implement automated workflows for subsurface analysis.
Advances in Subsurface Data Analytics: Traditional and Physics-Based Approaches will help researchers in academia and professional geoscientists working on the subsurface-related problems (oil and gas, geothermal, carbon sequestration, and seismology) at different scales to understand and appreciate current trends in ML approaches, their applications, advances and limitations, and future potential in geosciences by bringing together several contributions in a single volume.
- Covers fundamentals of simple machine learning and deep learning algorithms, and physics-based approaches written by practitioners in academia and industry
- Presents detailed case studies of individual machine learning algorithms and optimal strategies in subsurface characterization around the world
- Offers an analysis of future trends in machine learning in geosciences
- Cover Image
- Title Page
- Copyright
- Table of Contents
- Contributors
- About the Editors
- Preface
- Acknowledgments
- Part 1 Traditional machine learning approaches
- Chapter 1 User vs. machine-based seismic attribute selection for unsupervised machine learning techniques: Does human insight provide better results than statistically chosen attributes?
- 1.1 Introduction
- 1.2 Motivation
- 1.3 Dataset characteristics
- 1.4 Seismic attributes
- 1.5 Principal component analysis (PCA)
- 1.6 Self-organizing maps (SOMs): An unsupervised technique for seismic facies identification
- 1.7 Methodology
- 1.8 Results
- 1.9 Self-organizing maps analysis
- 1.10 SOM results and discussion from user-selected attributes vs. machine-derived inputs
- 1.11 Human vs. machine comparison (upsides and downsides of PCA vs multiattribute analysis)
- 1.12 Recommendations
- 1.13 Conclusion
- 1.14 Acknowledgments
- References
- Chapter 2 Relative performance of support vector machine, decision trees, and random forest classifiers for predicting production success in US unconventional shale plays
- 2.1 Introduction
- 2.2 Methods
- 2.3 Results
- 2.4 Discussion
- 2.5 Conclusion
- 2.6 Acknowledgments
- References
- Part 2 Deep learning approaches
- Chapter 3 Recurrent neural network: application in facies classification
- 3.1 Introduction
- 3.2 Data types
- 3.3 Recurrent neural network (RNN) methods
- 3.4 Case study: Bi-LSTM-assisted facies classification based on well logging data
- 3.5 Results and discussion
- 3.6 Conclusion
- References
- Chapter 4 Recurrent neural network for seismic reservoir characterization
- 4.1 Introduction
- 4.2 Methodolgy
- 4.3 Applications
- 4.4 Conclusion
- References
- Chapter 5 Convolutional neural networks: core interpretation with instance segmentation models
- 5.1 Introduction
- 5.2 Methods
- 5.3 Results
- 5.4 Discussion
- 5.5 Conclusion
- 5.6 Acknowledgments
- References
- Chapter 6 Convolutional neural networks for fault interpretation – case study examples around the world
- 6.1 Introduction
- 6.2 Machine learning algorithms
- 6.3 Data and workflow
- 6.4 Case studies
- 6.5 Discussions
- 6.6 Conclusions
- 6.7 Acknowledgments
- References
- Part 3 Physics-based machine learning approaches
- Chapter 7 Applying scientific machine learning to improve seismic wave simulation and inversion
- 7.1 Introduction
- 7.2 Related work
- 7.3 Wave equations and RNN
- 7.4 Differentiable programming
- 7.5 Seismic inversion
- 7.6 Discussion
- 7.7 Conclusions
- 7.8 Acknowledgment
- References
- Chapter 8 Prediction of acoustic velocities using machine learning and rock physics
- 8.1 Introduction
- 8.2 Conventional rock physics modeling
- 8.3 Machine learning methods
- 8.4 Synthetic data test
- 8.5 Real data test
- 8.6 Discussion
- 8.7 Conclusions
- 8.8 Acknowledgments
- References
- Chapter 9 Regularized elastic full-waveform inversion using deep learning
- 9.1 Introduction
- 9.2 Methodology
- 9.3 Numerical examples
- 9.4 Discussion
- 9.5 Conclusions
- 9.6 Acknowledgments
- 9.7 Appendix example training code
- References
- Chapter 10 A holistic approach to computing first-arrival traveltimes using neural networks
- 10.1 Introduction
- 10.2 Theory
- 10.3 Numerical tests
- 10.4 Discussion and conclusions
- References
- Part 4 Physics-based machine learning approaches
- Chapter 11 Application of artificial intelligence to computational fluid dynamics
- 11.1 Introduction
- 11.2 Background
- 11.3 B6 combustor model
- 11.4 Smart proxy development steps
- 11.5 Conclusions
- 11.6 Appendix
- 11.7 Acknowledgments
- 11.8 Disclaimer
- References
- Index
- Edition: 1
- Published: May 18, 2022
- Imprint: Elsevier
- No. of pages: 376
- Language: English
- Paperback ISBN: 9780128222959
- eBook ISBN: 9780128223086
SB
Shuvajit Bhattacharya
HD