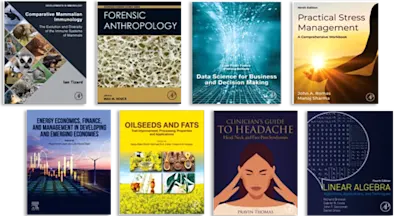
Advances in Streamflow Forecasting
From Traditional to Modern Approaches
- 1st Edition - June 20, 2021
- Imprint: Elsevier
- Editors: Priyanka Sharma, Deepesh Machiwal
- Language: English
- Paperback ISBN:9 7 8 - 0 - 1 2 - 8 2 0 6 7 3 - 7
- eBook ISBN:9 7 8 - 0 - 1 2 - 8 2 0 9 2 4 - 0
Advances in Streamflow Forecasting: From Traditional to Modern Approaches covers the three major data-driven approaches of streamflow forecasting including traditional approach of… Read more
Purchase options
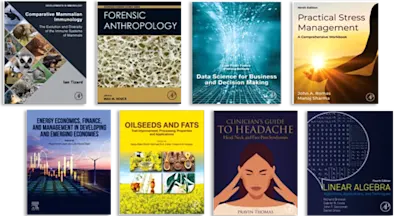
Institutional subscription on ScienceDirect
Request a sales quoteAdvances in Streamflow Forecasting: From Traditional to Modern Approaches covers the three major data-driven approaches of streamflow forecasting including traditional approach of statistical and stochastic time-series modelling with their recent developments, stand-alone data-driven approach such as artificial intelligence techniques, and modern hybridized approach where data-driven models are combined with preprocessing methods to improve the forecast accuracy of streamflows and to reduce the forecast uncertainties.
This book starts by providing the background information, overview, and advances made in streamflow forecasting. The overview portrays the progress made in the field of streamflow forecasting over the decades. Thereafter, chapters describe theoretical methodology of the different data-driven tools and techniques used for streamflow forecasting along with case studies from different parts of the world. Each chapter provides a flowchart explaining step-by-step methodology followed in applying the data-driven approach in streamflow forecasting.
This book addresses challenges in forecasting streamflows by abridging the gaps between theory and practice through amalgamation of theoretical descriptions of the data-driven techniques and systematic demonstration of procedures used in applying the techniques. Language of this book is kept simple to make the readers understand easily about different techniques and make them capable enough to straightforward replicate the approach in other areas of their interest.
This book will be vital for hydrologists when optimizing the water resources system, and to mitigate the impact of destructive natural disasters such as floods and droughts by implementing long-term planning (structural and nonstructural measures), and short-term emergency warning. Moreover, this book will guide the readers in choosing an appropriate technique for streamflow forecasting depending upon the given set of conditions.
- Contributions from renowned researchers/experts of the subject from all over the world to provide the most authoritative outlook on streamflow forecasting
- Provides an excellent overview and advances made in streamflow forecasting over the past more than five decades and covers both traditional and modern data-driven approaches in streamflow forecasting
- Includes case studies along with detailed flowcharts demonstrating a systematic application of different data-driven models in streamflow forecasting, which helps understand the step-by-step procedures
Forecasters, Watershed Scientists, Natural Resource/Water Managers, Meteorologists, Civil Engineers, Statisticians
Priyanka Sharma and Deepesh Machiwal
2. Streamflow Forecasting at Large Time Scales Using Statistical Models
Hristos Tyralis, Georgia Papacharalampous and Andreas Langousis
3. Introduction of Multiple/Multivariate Linear and Nonlinear Time Series Models in Forecasting Streamflow Process
Farshad Fathian
4. Concepts and Procedures of Artificial Neural Network Models for Streamflow Forecasting
Arash Malekian and Nastaran Chitsaz
5. Application of Different Artificial Neural Network Models in Streamflow Forecasting
Md. Manjurul Hussain, Sheikh Hefzul Bari, Ishtiak Mahmud, Mohammad Istiyak Hossain Siddiquee
6. Application of Artificial Neural Network Model and Adaptive Neuro-Fuzzy Inference System in Streamflow Forecasting
Mehdi Vafakhah and Saeid Janizadeh
7. Genetic Programming for Streamflow Forecasting: A Concise Review of Univariate Models with a Case Study
Ali Danandeh Mehr and Mir Jafar Sadegh Safari
8. Model Tree Technique for Streamflow Forecasting: A Case Study of a Sub-catchment in Tapi River Basin, India
Priyank J. Sharma, P. L. Patel and V. Jothiprakash
9. Averaging Multi-climate Model Prediction of Streamflow in the Machine Learning Paradigm
Kevin O. Achieng
10. Short-term Flood Forecasting using Artificial Neural Network, Extreme Learning Machines and M5 Tree Models
Mukesh K. Tiwari, Ravinesh C. Deo and Jan F. Adamowski
11. A New Heuristic Method for Monthly Streamflow Forecasting: Outlier-Robust Extreme Learning Machine
Salim Heddam and Özgur Kişi
12. Hybrid Artificial Intelligence Models for Predicting Daily Runoff
Anurag Malik, Anil Kumar, Yazid Tikhamarine, Doudja Souag-Gamane and Özgur Kişi
13. Flood Forecasting and Error Simulation Using Copula and Entropy Methods
Lu Chen and Vijay P. Singh
- Edition: 1
- Published: June 20, 2021
- No. of pages (Paperback): 404
- Imprint: Elsevier
- Language: English
- Paperback ISBN: 9780128206737
- eBook ISBN: 9780128209240
PS
Priyanka Sharma
DM