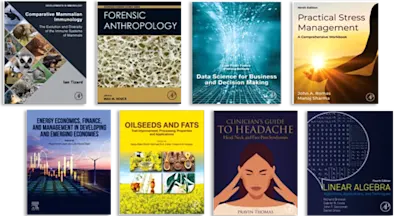
Acoustic Emission Signal Analysis and Damage Mode Identification of Composite Wind Turbine Blades
- 1st Edition - May 20, 2023
- Imprint: Elsevier
- Author: Pengfei Liu
- Language: English
- Paperback ISBN:9 7 8 - 0 - 3 2 3 - 8 8 6 5 2 - 9
- eBook ISBN:9 7 8 - 0 - 3 2 3 - 9 5 8 1 3 - 4
- eBook ISBN:9 7 8 - 0 - 3 2 3 - 8 8 6 4 7 - 5
Acoustic Emission Signal Analysis and Damage Mode Identification of Composite Wind Turbine Blades covers both the underlying theory and various techniques for effective structura… Read more
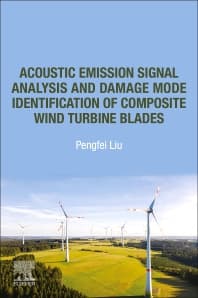
Purchase options
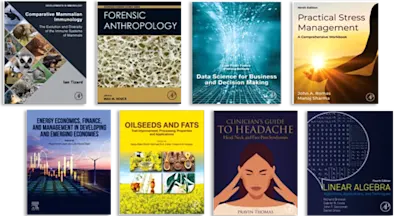
Institutional subscription on ScienceDirect
Request a sales quoteAcoustic Emission Signal Analysis and Damage Mode Identification of Composite Wind Turbine Blades covers both the underlying theory and various techniques for effective structural monitoring of composite wind turbine blades via acoustic emission signal analysis, helping readers solve critical problems such as noise elimination, defect detection, damage mode identification, and more. Author Pengfei Liu introduces techniques for identifying and analyzing progressive failure under tension, delamination, damage localization, adhesive composite joint failure, and other degradation phenomena, outlining methods such as time-difference, wavelet, machine learning, and more including combined methods.
The disadvantages and advantages of using each method are covered as are techniques for different blade-lengths and various blade substructures. Piezoelectric sensors are discussed as is experimental analysis of damage source localization. The book also takes great lengths to let readers know when techniques and concepts discussed can be applied to composite materials and structures beyond just wind turbine blades.
- Features fundamental acoustic emission theories and techniques for monitoring the structural integrity of wind turbine blades
- Covers sensor arrangements, noise elimination, defect detection, and dominating damage mode identification using acoustic emission techniques
- Outlines the wavelet method, the time-difference defect detection method, and damage mode identification techniques using machine learning
- Discusses how the techniques covered can be extended and adapted for use in other composite structures under complex loads and in different environments
Advanced undergraduate and graduate students;
- Cover image
- Title page
- Table of Contents
- Copyright
- Preface
- 1. AE health monitoring technique for composite wind turbine blade: a state-of-art review
- 1.1. Basic structures and damage mechanisms of wind turbine blades
- 1.2. Structural health monitoring techniques of wind turbine blades
- 1.3. Basic principles and feature parameters of AE technique
- 1.4. Defect positioning techniques using AE signals
- 1.5. Damage mode identification methods using AE signals
- 1.6. Damage evaluation models using AE signals
- 1.7. Concluding remarks
- 2. AE tests and signal analysis of composite laminates with a central hole under tension
- 2.1. AE tests on composite laminates under tension
- 2.2. AE signal analysis
- 2.3. Concluding remarks
- 3. AE tests and signal analysis on delamination of composite laminates under three-point bending and different temperature
- 3.1. Delamination experiments and AE tests of composite laminates
- 3.2. Mechanical properties of composite laminates
- 3.3. AE signal analysis of delamination failure of composite laminates
- 3.4. Concluding remarks
- 4. AE tests and signal analysis on delamination of composite laminates under three-point bending and hygrothermal environments
- 4.1. Delamination experiments and AE tests of composite laminates under hygrothermal environment
- 4.2. AE signal analysis of delamination failure of composite laminates under hygrothermal environment
- 4.3. AE spectrum analysis of delamination failure of composite laminates under hygrothermal environment
- 4.4. Concluding remarks
- 5. AE tests and signal analysis of composite laminates under tensile strain rate loads
- 5.1. Single-leg bending tests and AE analysis for delamination of composite laminates
- 5.2. Concluding remarks
- 6. AE tests and signal analysis on Delamination of composite laminates under tension–tension fatigue loads
- 6.1. Tension–tension fatigue loads of unidirectional composite laminates
- 6.2. AE signal analysis for composite laminates under fatigue loads
- 6.3. Concluding remarks
- 7. AE tests and damage mode identification of composite wind turbine blade under bending fatigue loads
- 7.1. AE tests of wind turbine blade under fatigue loads
- 7.2. AE signal analysis
- 7.3. Concluding remarks
- 8. A waveform-based feature extraction model for AE signal analysis for structural health monitoring of composite wind turbine blade
- 8.1. Methodology for AE signal analysis of wind turbine blade under fatigue loads
- 8.2. Results and discussion
- 8.3. An alarm index for failure prevention of blade
- 8.4. Concluding remarks
- 9. Damage mode identification of composite materials based on global AE data and clustering analysis
- 9.1. AE features of different artificially excited sound sources
- 9.2. AE tests of double-lap composite specimens under tensile loads
- 9.3. Feature selection methods
- 9.4. Clustering algorithm by fast search and find of density peaks
- 9.5. Results and discussion
- 9.6. Concluding remarks
- 10. AE feature extraction and signal representation model based on wavelet packet decomposition
- 10.1. AE tests of composite unidirectional laminates under tensile load
- 10.2. AE feature extraction and signal characterization model
- 10.3. Damage mode identification of unidirectional composite laminates based on feature extraction
- 10.4. Damage behaviors of unidirectional composite laminates using AE
- 10.5. Concluding remarks
- 11. Dynamic feature evaluation and information mining for AE data stream
- 11.1. AE tests of adhesive composite single lap joints under tensile load
- 11.2. AE data flow methods
- 11.3. Dynamic evaluation of traditional AE features
- 11.4. Concluding remarks
- 12. Prediction model of residual load-bearing capacity of composite laminates using deep learning
- 12.1. AE tests of ENF composite laminates under bending loads
- 12.2. Prediction of residual load-bearing capacity of laminates based on feature evaluation and deep learning
- 12.3. Results and discussion
- 12.4. Mechanical state estimation of composite laminates based on modified Mel cepstrum analysis
- 12.5. Concluding remarks
- Index
- Edition: 1
- Published: May 20, 2023
- No. of pages (Paperback): 364
- No. of pages (eBook): 364
- Imprint: Elsevier
- Language: English
- Paperback ISBN: 9780323886529
- eBook ISBN: 9780323958134
- eBook ISBN: 9780323886475
PL